The Evolution of Artificial Intelligence: From Concept to Reality
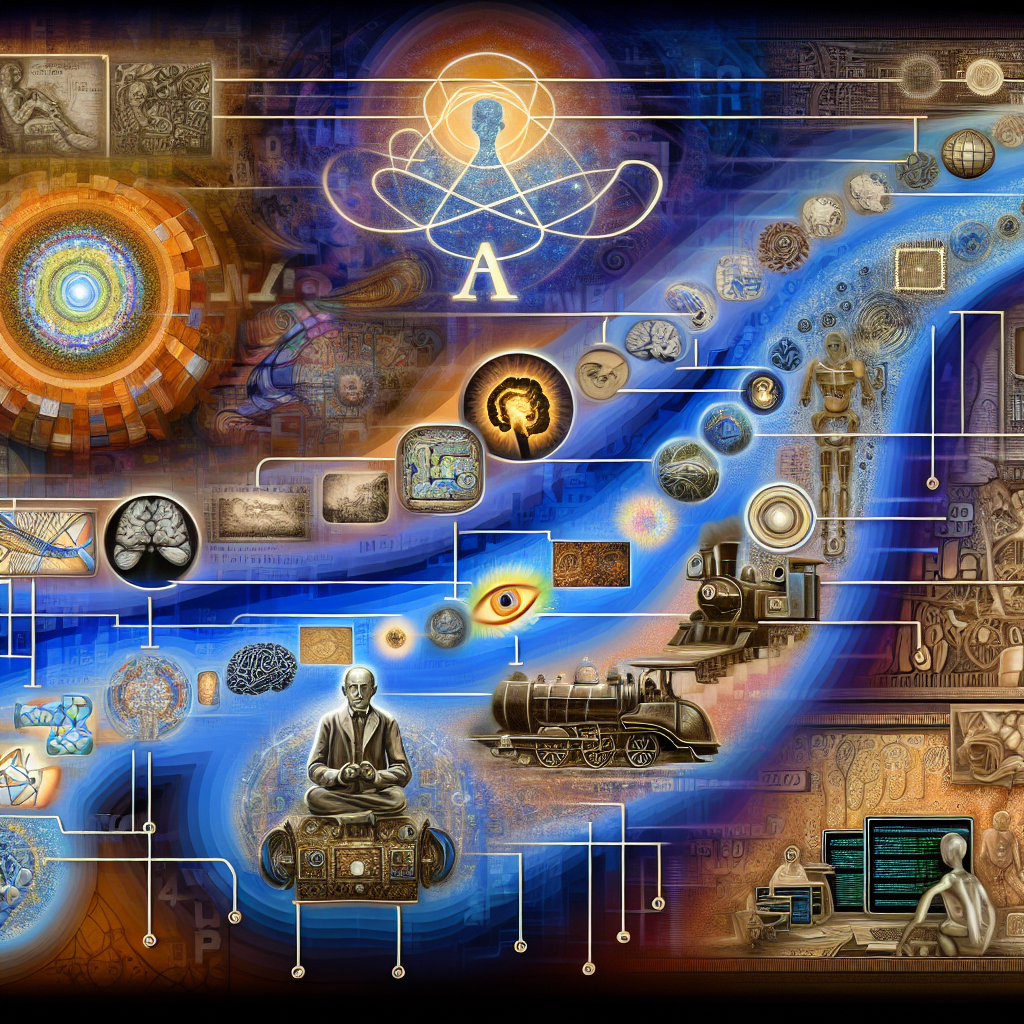
Table of Contents
Introduction
Artificial Intelligence (AI) has long been a subject of fascination and intrigue, captivating the minds of scientists, researchers, and the general public alike. From its early conceptualization in the mid-20th century to its current status as a transformative force in various industries, AI has come a long way. This article delves into the evolution of AI, tracing its journey from theoretical ideas to practical, real-world applications.
The Early Days of AI
The concept of artificial intelligence can be traced back to ancient civilizations, where myths and legends spoke of artificial beings endowed with intelligence. However, the formal foundation of AI as a scientific discipline began in the 1950s. Alan Turing, a British mathematician, proposed the idea of a ‘universal machine’ capable of performing computations similar to a human mind. This laid the groundwork for the development of the first AI programs.
The Dartmouth Conference
In 1956, the Dartmouth Conference marked a significant milestone in the history of AI. Organized by John McCarthy, Marvin Minsky, Nathaniel Rochester, and Claude Shannon, this conference brought together leading researchers to discuss the potential of creating machines that could ’think.’ It was during this conference that the term ‘Artificial Intelligence’ was coined. The event spurred a wave of optimism and research funding, leading to the creation of early AI programs capable of solving algebra problems, proving geometric theorems, and playing games like chess and checkers.
The Era of Symbolic AI
The 1960s and 1970s were dominated by symbolic AI, also known as ‘Good Old-Fashioned Artificial Intelligence’ (GOFAI). Researchers focused on developing systems that used symbolic representations of problems and logic-based approaches to solve them. Expert systems, which could mimic the decision-making abilities of human experts in specific domains, became popular during this period. Despite their initial success, these systems were limited by their reliance on explicitly defined rules and struggled with tasks requiring common sense and real-world knowledge.
The AI Winter
By the 1980s, the limitations of symbolic AI became apparent, leading to a period known as the ‘AI Winter.’ Funding for AI research dwindled as the initial optimism gave way to skepticism and disappointment. The inability of AI systems to handle uncertainties and the complexities of real-world environments contributed to this decline. However, this period also saw the emergence of new approaches, such as neural networks and machine learning, which would later revolutionize the field.
The Rise of Machine Learning
The 1990s and 2000s witnessed a resurgence of interest in AI, driven by advances in machine learning. Unlike symbolic AI, machine learning algorithms could learn from data and improve their performance over time. This shift from rule-based systems to data-driven approaches enabled AI to tackle a broader range of problems. Techniques like supervised learning, unsupervised learning, and reinforcement learning became fundamental to the development of intelligent systems.
The Age of Deep Learning
The 2010s marked the advent of deep learning, a subset of machine learning inspired by the structure and function of the human brain. Deep learning algorithms, particularly neural networks with multiple layers, demonstrated remarkable capabilities in tasks such as image and speech recognition, natural language processing, and autonomous driving. The availability of large datasets and powerful computational resources fueled the rapid progress of deep learning, making it a cornerstone of modern AI.
AI in the Real World
Today, AI has permeated various aspects of our lives, from virtual assistants like Siri and Alexa to recommendation systems on platforms like Netflix and Amazon. In healthcare, AI is being used for diagnosing diseases, predicting patient outcomes, and personalizing treatment plans. In finance, AI algorithms analyze market trends and detect fraudulent activities. The integration of AI in industries such as manufacturing, transportation, and entertainment continues to drive innovation and efficiency.
The Future of AI
As we look to the future, the potential of AI appears boundless. Researchers are exploring areas like explainable AI, which aims to make AI systems more transparent and understandable to humans. The ethical implications of AI, including issues of bias, privacy, and accountability, are also gaining attention. The development of artificial general intelligence (AGI), capable of performing any intellectual task that a human can, remains a long-term goal. While challenges and uncertainties lie ahead, the ongoing evolution of AI promises to reshape our world in profound ways.
In conclusion, the journey of artificial intelligence from its early conceptualization to its current state has been marked by significant milestones and transformative advancements. From the initial optimism of the Dartmouth Conference to the challenges of the AI Winter, and the resurgence brought about by machine learning and deep learning, AI has continuously evolved. As we stand on the cusp of new breakthroughs, the future of AI holds immense potential to revolutionize various aspects of our lives, making it an exciting field to watch and explore.