Machine Learning in IoT
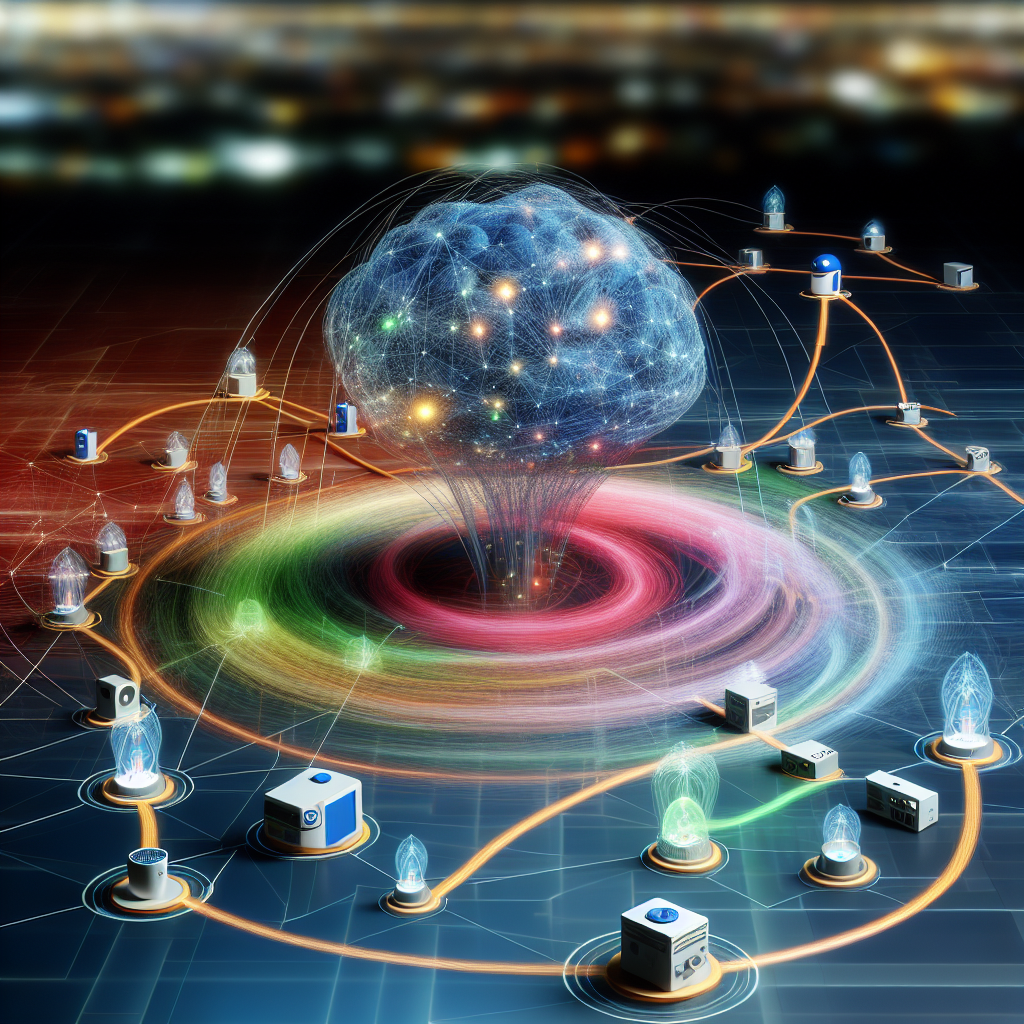
Table of Contents
Introduction to IoT
The Internet of Things (IoT) refers to the network of interconnected devices that communicate and exchange data with each other. These devices, ranging from household appliances to industrial machinery, are embedded with sensors, software, and other technologies to collect and transmit data. The IoT ecosystem enables smarter living and more efficient operations across various sectors, such as healthcare, transportation, and manufacturing.
The Role of Machine Learning in IoT
Machine learning (ML) plays a crucial role in enhancing the capabilities of IoT devices. By leveraging algorithms that can learn from data, ML enables IoT systems to make intelligent decisions and predictions. This integration helps in processing vast amounts of data generated by IoT devices, identifying patterns, and automating responses. As a result, IoT systems become more efficient, adaptive, and capable of delivering personalized experiences.
Benefits of Machine Learning in IoT
The combination of machine learning and IoT brings numerous benefits. Firstly, it improves predictive maintenance by analyzing data from sensors to predict equipment failures before they occur. This reduces downtime and maintenance costs. Secondly, ML enhances security by detecting anomalies and potential threats in real-time, thereby safeguarding sensitive data. Additionally, it enables personalized user experiences by analyzing behavior patterns and preferences, which can be applied in smart homes, healthcare, and retail.
Challenges in Integrating Machine Learning with IoT
Despite its potential, integrating machine learning with IoT faces several challenges. One of the significant issues is data privacy and security, as IoT devices often handle sensitive information. Ensuring robust encryption and secure data transmission is crucial. Another challenge is the computational power required for ML algorithms, which can be demanding for some IoT devices. Moreover, the heterogeneity of IoT devices and the lack of standardization can hinder seamless integration and interoperability.
Applications of Machine Learning in IoT
Machine learning enhances various IoT applications. In healthcare, ML algorithms analyze data from wearable devices to monitor patient health and predict potential issues. In smart cities, it optimizes traffic flow and energy consumption by analyzing data from sensors and cameras. In manufacturing, ML improves quality control and predictive maintenance by analyzing production data. Additionally, in agriculture, ML analyzes data from sensors to optimize irrigation, monitor crop health, and increase yield.
Future Prospects of Machine Learning in IoT
The future of machine learning in IoT is promising, with advancements in AI and data analytics driving innovation. As IoT devices become more sophisticated, the integration of ML will enable even more intelligent and autonomous systems. Edge computing, where data processing occurs closer to the data source, will further enhance the efficiency and responsiveness of IoT systems. Furthermore, the development of federated learning will allow IoT devices to collaborate and learn from each other while maintaining data privacy.
In conclusion, the synergy between machine learning and IoT is transforming various industries by enabling smarter, more efficient, and adaptive systems. While there are challenges to overcome, the potential benefits far outweigh the obstacles. As technology continues to evolve, the integration of machine learning in IoT will play a pivotal role in shaping the future of connected devices and smart systems.