Understanding Bayesian Statistics
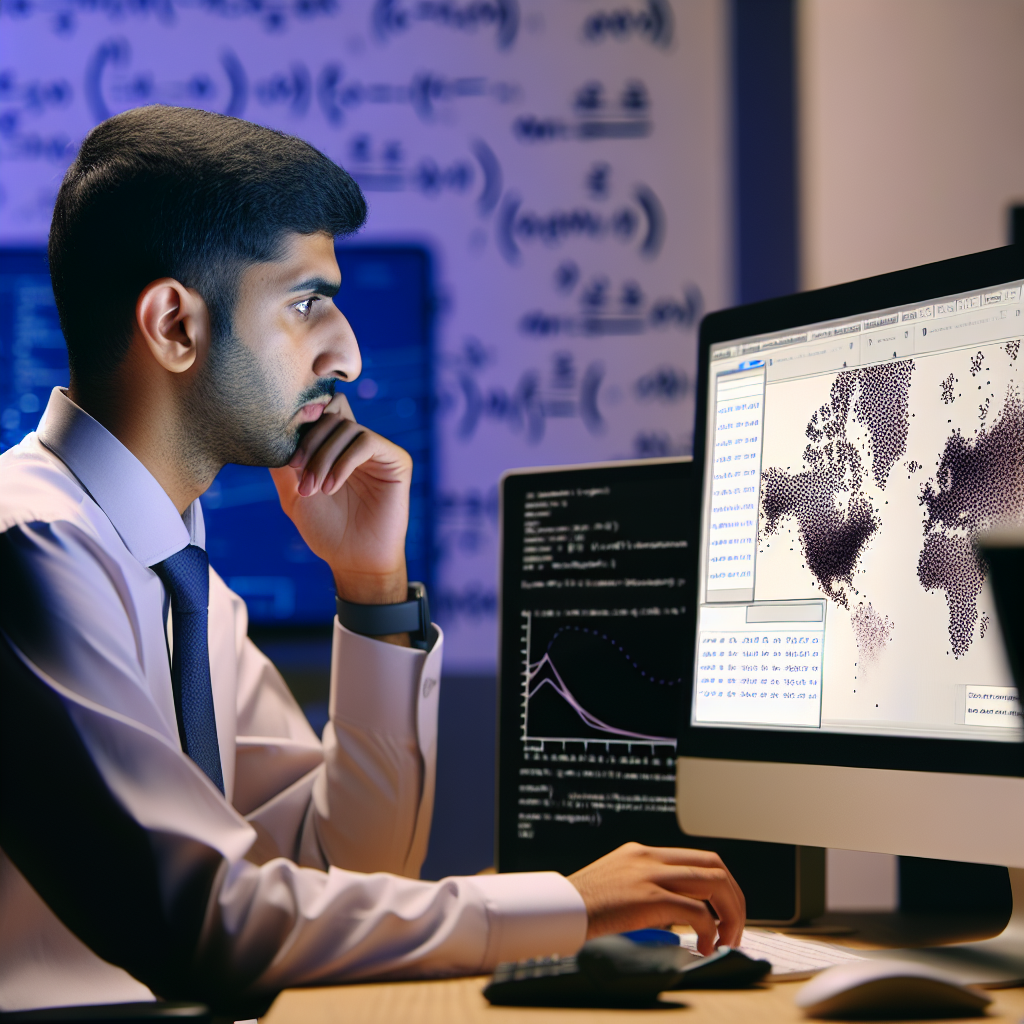
Table of Contents
Introduction
Bayesian statistics is a powerful and flexible approach to statistical analysis that has gained significant attention and application in various fields. Unlike classical statistics, which relies on frequency-based interpretations of probability, Bayesian statistics incorporates prior knowledge and evidence to update the probability of a hypothesis as new data becomes available. This article delves into the principles, applications, and impact of Bayesian statistics on modern data analysis.
The Core Principle of Bayesian Statistics
At the heart of Bayesian statistics lies Bayes’ theorem, a fundamental principle that describes how to update the probability of a hypothesis in light of new evidence. Bayes’ theorem is mathematically expressed as: P(H|E) = [P(E|H) * P(H)] / P(E), where P(H|E) is the posterior probability, P(E|H) is the likelihood, P(H) is the prior probability, and P(E) is the marginal likelihood. This formula allows statisticians to combine prior beliefs with new data to make informed inferences.
Prior, Likelihood, and Posterior
Understanding the components of Bayes’ theorem is crucial for grasping Bayesian statistics. The ‘prior’ represents the initial belief about a hypothesis before any new data is considered. The ’likelihood’ indicates the probability of observing the new data given the hypothesis. The ‘posterior’ is the updated probability of the hypothesis after considering the new data. This iterative process of updating beliefs is what makes Bayesian statistics particularly powerful in dynamic and uncertain environments.
Applications of Bayesian Statistics
Bayesian statistics has found applications across a wide range of disciplines, from medicine and genetics to machine learning and finance. In medicine, Bayesian methods are used to update the probability of disease presence based on diagnostic test results and prior information about the patient. In genetics, Bayesian approaches help in mapping genes associated with diseases by integrating prior biological knowledge with experimental data. Machine learning models often employ Bayesian techniques to improve predictions and handle uncertainty. In finance, Bayesian methods assist in portfolio optimization and risk assessment by incorporating prior market information and new financial data.
Advantages of Bayesian Statistics
One of the primary advantages of Bayesian statistics is its ability to incorporate prior knowledge into the analysis. This is particularly beneficial in fields where historical data or expert knowledge is available. Bayesian methods also provide a coherent framework for updating beliefs in the face of new evidence, making them well-suited for dynamic environments. Additionally, Bayesian statistics offer a more intuitive interpretation of probability as a degree of belief, which can be more meaningful in real-world decision-making compared to the frequency-based approach of classical statistics.
Challenges and Criticisms
Despite its advantages, Bayesian statistics is not without its challenges and criticisms. One common criticism is the subjective nature of choosing prior distributions, which can influence the results of the analysis. Additionally, Bayesian methods can be computationally intensive, especially for complex models and large datasets. Critics also argue that the reliance on prior knowledge may introduce biases if the priors are not appropriately chosen. Nevertheless, advancements in computational techniques and the development of objective priors have addressed some of these concerns, making Bayesian statistics more accessible and robust.
Conclusion
Bayesian statistics represents a paradigm shift in the way we approach statistical inference and data analysis. By integrating prior knowledge with new data, it offers a flexible and dynamic framework for making informed decisions under uncertainty. While there are challenges and criticisms associated with Bayesian methods, their advantages and widespread applications across various fields underscore their importance in modern statistical practice. As computational capabilities continue to advance, the role of Bayesian statistics is likely to expand, further transforming the landscape of data analysis and decision-making.