Statistical Inference
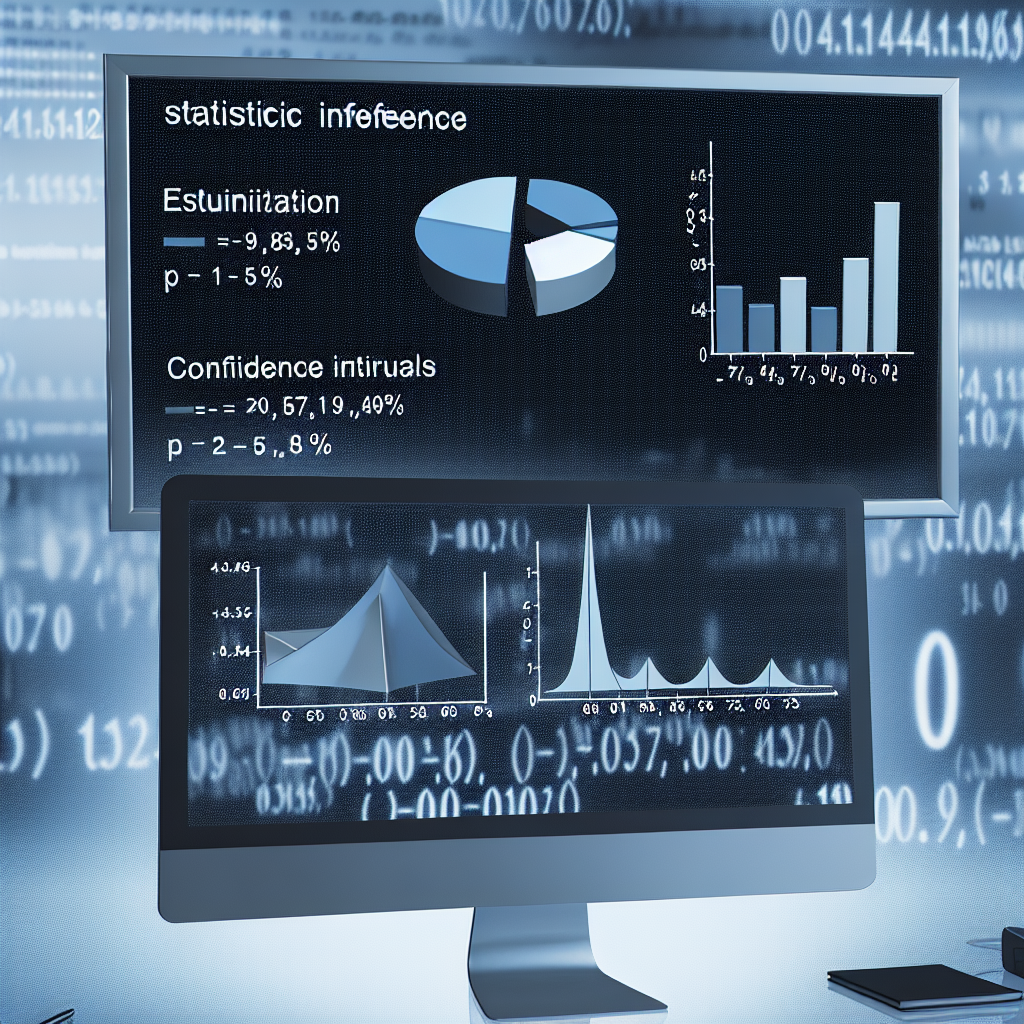
Table of Contents
Introduction
Statistical inference is a fundamental aspect of statistics that involves drawing conclusions about a population based on a sample of data taken from that population. It uses probability theory to estimate population parameters, test hypotheses, and make predictions. This process is crucial for making informed decisions in various fields such as economics, medicine, social sciences, and engineering.
The Importance of Statistical Inference
The significance of statistical inference lies in its ability to provide a framework for making decisions under uncertainty. By analyzing sample data, statisticians can infer properties about a larger population. This is particularly useful when it is impractical or impossible to collect data from every member of a population. For instance, in clinical trials, it is not feasible to test a new drug on every patient, so a sample is used to infer the drug’s effectiveness.
Types of Statistical Inference
There are two main types of statistical inference: estimation and hypothesis testing. Estimation involves determining the approximate value of a population parameter based on sample data. Hypothesis testing, on the other hand, involves making decisions about the population by testing assumptions or claims. Both methods rely on the principles of probability theory to make valid inferences.
Estimation
Estimation can be further divided into point estimation and interval estimation. Point estimation provides a single value as an estimate of a population parameter. For example, the sample mean is often used as a point estimate of the population mean. Interval estimation, however, provides a range of values within which the population parameter is expected to lie. This range is typically expressed with a confidence level, such as a 95% confidence interval, which indicates that there is a 95% probability that the interval contains the population parameter.
Hypothesis Testing
Hypothesis testing is a method for testing a claim or hypothesis about a population parameter. It involves formulating a null hypothesis (H0) and an alternative hypothesis (H1). The null hypothesis represents a statement of no effect or no difference, while the alternative hypothesis represents the effect or difference being tested. Statistical tests are then used to determine whether there is enough evidence to reject the null hypothesis in favor of the alternative hypothesis. Common tests include the t-test, chi-square test, and ANOVA.
P-values and Significance Levels
A key concept in hypothesis testing is the p-value, which measures the probability of obtaining the observed sample results, or more extreme results, if the null hypothesis is true. The significance level (alpha) is a threshold set by the researcher, typically 0.05, which determines whether the p-value is low enough to reject the null hypothesis. If the p-value is less than the significance level, the null hypothesis is rejected, suggesting that the sample provides sufficient evidence to support the alternative hypothesis.
Assumptions in Statistical Inference
Statistical inference relies on certain assumptions about the data and the population from which it is drawn. Common assumptions include randomness of the sample, independence of observations, and the normality of the population distribution. Violations of these assumptions can lead to incorrect conclusions. Therefore, it is important to assess the validity of these assumptions before applying statistical inference methods.
Applications of Statistical Inference
Statistical inference is widely used in various fields to make informed decisions based on data. In healthcare, it is used to evaluate the effectiveness of treatments and interventions. In economics, it helps in making forecasts and policy decisions. In engineering, it is used for quality control and reliability testing. The versatility and reliability of statistical inference make it an indispensable tool in data analysis and decision-making.
Conclusion
In conclusion, statistical inference is a powerful method for drawing conclusions about a population based on sample data. Through estimation and hypothesis testing, it allows researchers and practitioners to make informed decisions under uncertainty. Understanding the principles and assumptions of statistical inference is essential for correctly applying these methods and interpreting the results. As data continues to play a crucial role in various domains, the importance of statistical inference in data-driven decision-making cannot be overstated.