Statistical Hypothesis Testing
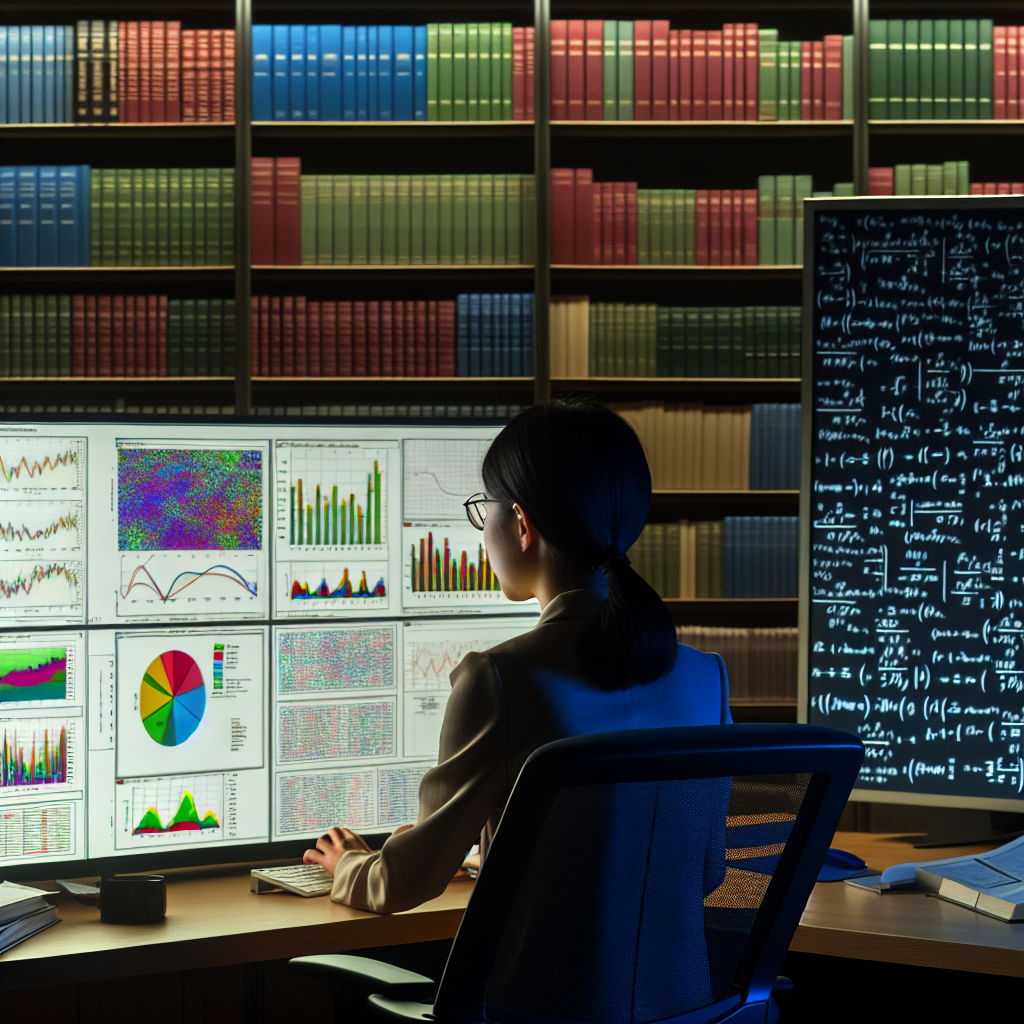
Table of Contents
Introduction to Statistical Hypothesis Testing
Statistical hypothesis testing is a fundamental aspect of statistical inference that allows researchers to make decisions or inferences about a population based on sample data. It is a method used to test an assumption regarding a population parameter. The process involves formulating two competing hypotheses: the null hypothesis (H0) and the alternative hypothesis (H1). The null hypothesis represents a statement of no effect or no difference, while the alternative hypothesis represents the statement that there is an effect or a difference.
The Null and Alternative Hypotheses
The null hypothesis (H0) is a statement that indicates no effect or no difference in the population. It is the hypothesis that researchers aim to test against. The alternative hypothesis (H1), on the other hand, is a statement that indicates the presence of an effect or a difference. The goal of hypothesis testing is to determine whether there is enough evidence in the sample data to reject the null hypothesis in favor of the alternative hypothesis. It is important to note that rejecting the null hypothesis does not prove the alternative hypothesis; it merely suggests that there is sufficient evidence to support it.
Types of Hypothesis Tests
Hypothesis tests can be classified into several types based on the nature of the data and the specific questions being addressed. Some common types of hypothesis tests include the t-test, chi-square test, ANOVA (Analysis of Variance), and the z-test. Each of these tests has specific conditions and assumptions that must be met for the test results to be valid. For example, the t-test is used to compare the means of two groups, while the chi-square test is used to test the association between categorical variables. ANOVA is used to compare the means of three or more groups, and the z-test is used when the sample size is large and the population variance is known.
The P-Value and Significance Level
The p-value is a crucial component of hypothesis testing. It represents the probability of obtaining the observed sample results, or more extreme results, assuming that the null hypothesis is true. A smaller p-value indicates stronger evidence against the null hypothesis. Researchers typically compare the p-value to a predetermined significance level (alpha), which is usually set at 0.05. If the p-value is less than the significance level, the null hypothesis is rejected in favor of the alternative hypothesis. The significance level represents the probability of making a Type I error, which is the incorrect rejection of a true null hypothesis.
Type I and Type II Errors
In statistical hypothesis testing, there are two types of errors that researchers must be aware of: Type I and Type II errors. A Type I error occurs when the null hypothesis is incorrectly rejected when it is actually true. The probability of making a Type I error is denoted by the significance level (alpha). A Type II error occurs when the null hypothesis is not rejected when it is actually false. The probability of making a Type II error is denoted by beta. Researchers aim to minimize both types of errors, but there is often a trade-off between them. Reducing the significance level reduces the probability of a Type I error but increases the probability of a Type II error, and vice versa.
Applications of Hypothesis Testing
Statistical hypothesis testing is widely used in various fields of research, including medicine, psychology, economics, and social sciences. In medicine, hypothesis testing is used to determine the effectiveness of new treatments or drugs. In psychology, it is used to test theories about human behavior and cognitive processes. In economics, hypothesis testing is used to analyze economic trends and relationships. In social sciences, it is used to study social phenomena and relationships between variables. The versatility and robustness of hypothesis testing make it an invaluable tool for researchers across disciplines.
Conclusion
Statistical hypothesis testing is a powerful method for making inferences about population parameters based on sample data. By formulating and testing hypotheses, researchers can draw conclusions about the presence or absence of effects and differences in the population. Understanding the principles of hypothesis testing, including the null and alternative hypotheses, types of tests, p-values, and significance levels, is essential for conducting rigorous and valid research. Despite its complexities, hypothesis testing remains a cornerstone of statistical analysis and continues to play a critical role in advancing knowledge across various fields.