Multivariate Analysis
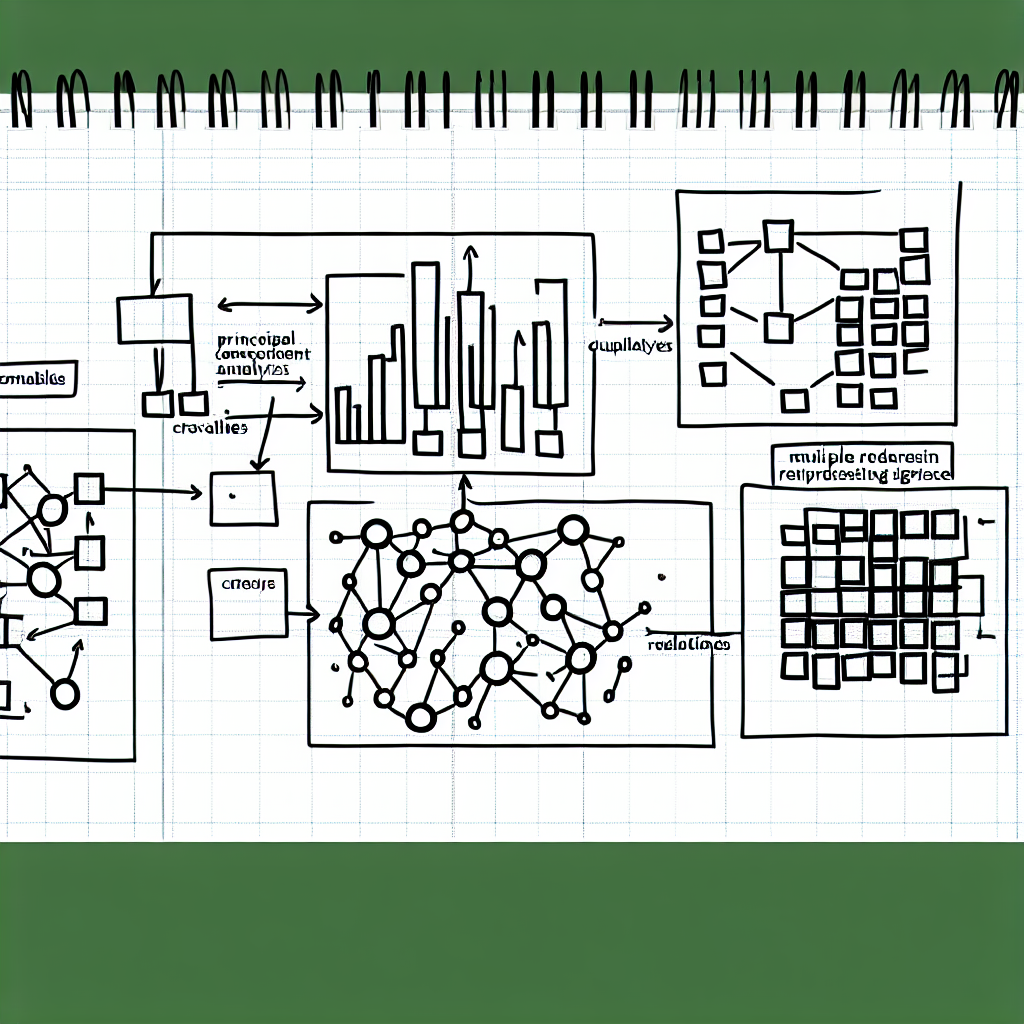
Table of Contents
Introduction
Multivariate analysis is a powerful statistical tool used to understand complex data sets involving multiple variables. Unlike univariate or bivariate analysis, which consider one or two variables respectively, multivariate analysis examines more than two variables simultaneously. This approach allows for a more comprehensive understanding of the relationships between variables and the underlying structure of the data.
Types of Multivariate Analysis
There are several types of multivariate analysis techniques, each designed to address specific types of research questions and data structures. Some of the most commonly used techniques include Principal Component Analysis (PCA), Factor Analysis, Cluster Analysis, and Multiple Regression Analysis. Each of these methods has its own unique applications and assumptions, making them suitable for different types of data and research objectives.
Principal Component Analysis (PCA)
Principal Component Analysis (PCA) is a technique used to reduce the dimensionality of large data sets by transforming the original variables into a new set of uncorrelated variables called principal components. These principal components capture the maximum variance in the data, making it easier to visualize and interpret. PCA is widely used in fields such as genomics, finance, and image processing to identify patterns and simplify complex data structures.
Factor Analysis
Factor Analysis is another multivariate technique that aims to identify underlying factors or latent variables that explain the observed correlations among a set of observed variables. Unlike PCA, which is primarily a data reduction technique, Factor Analysis seeks to uncover the hidden structure in the data. This method is often used in psychology, marketing, and social sciences to identify underlying dimensions of constructs such as intelligence, consumer preferences, or social attitudes.
Cluster Analysis
Cluster Analysis is a technique used to group similar observations into clusters based on their characteristics. This method helps to identify natural groupings in the data and is commonly used in market segmentation, customer profiling, and image recognition. There are several algorithms for cluster analysis, including k-means, hierarchical clustering, and DBSCAN, each with its own strengths and limitations.
Multiple Regression Analysis
Multiple Regression Analysis is a statistical technique that models the relationship between a dependent variable and multiple independent variables. This method allows researchers to understand how changes in the independent variables affect the dependent variable and to make predictions based on the model. Multiple Regression is widely used in economics, medicine, and social sciences to analyze complex relationships and make informed decisions.
Applications of Multivariate Analysis
Multivariate analysis has a wide range of applications across various fields. In healthcare, it is used to analyze patient data and identify risk factors for diseases. In marketing, it helps businesses understand customer behavior and preferences. In finance, it is used to assess risk and optimize investment portfolios. The ability to analyze multiple variables simultaneously makes multivariate analysis a valuable tool for uncovering insights and making data-driven decisions.
Challenges and Considerations
While multivariate analysis offers many benefits, it also comes with challenges. One of the main challenges is the complexity of the data and the need for advanced statistical knowledge to perform and interpret the analysis correctly. Additionally, multicollinearity, which occurs when independent variables are highly correlated, can complicate the analysis and lead to misleading results. It is essential to carefully consider the assumptions and limitations of each technique and to validate the results through rigorous testing and validation.
Conclusion
In conclusion, multivariate analysis is a crucial component of modern data analysis, offering powerful tools to understand complex data sets and uncover hidden relationships. By leveraging techniques such as PCA, Factor Analysis, Cluster Analysis, and Multiple Regression, researchers and analysts can gain valuable insights and make informed decisions. Despite its challenges, the benefits of multivariate analysis make it an indispensable tool in the era of big data and advanced analytics.