Factor Analysis
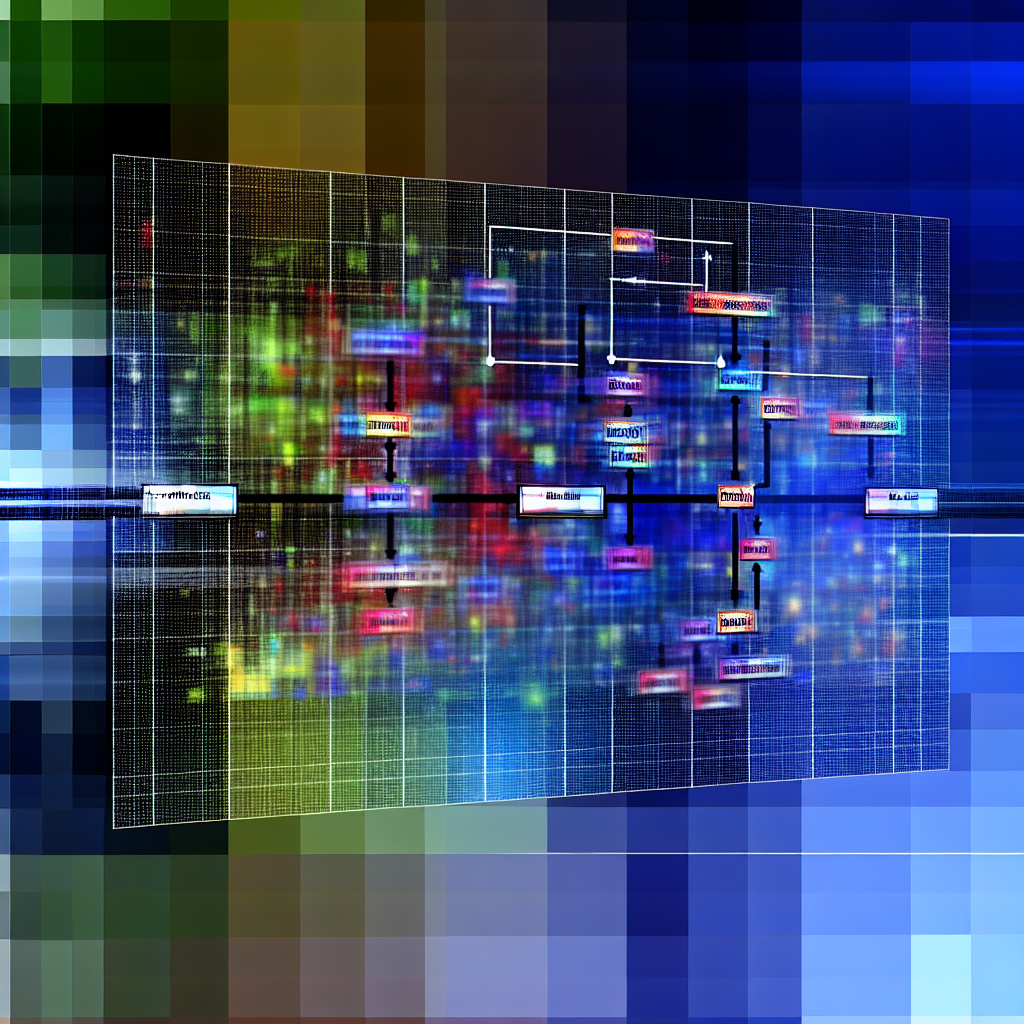
Table of Contents
Introduction
Factor analysis is a statistical method used primarily in the fields of psychology, social sciences, marketing, and other domains that deal with large datasets. It aims to identify underlying relationships between observed variables by reducing the number of variables into fewer, unobserved variables known as factors. This technique helps in simplifying complex data structures and is particularly useful in the development of theories and models.
Historical Background
The roots of factor analysis can be traced back to the early 20th century. Charles Spearman, a pioneering figure in the field of psychology, introduced the concept of factor analysis in 1904. He used this method to study human intelligence, proposing that a single general intelligence factor, ‘g’, could explain the correlations among different cognitive tasks. Over the years, the methodology has evolved, with significant contributions from other statisticians and psychologists, leading to more robust and versatile techniques.
Types of Factor Analysis
There are mainly two types of factor analysis: Exploratory Factor Analysis (EFA) and Confirmatory Factor Analysis (CFA). EFA is used when the researcher does not have a priori hypotheses about the underlying structure of the data. It is a data-driven approach that helps in identifying the possible number of factors and their loadings. On the other hand, CFA is hypothesis-driven and is used to test the validity of a pre-specified factor structure. It involves specifying the number of factors and the variables that load on each factor before analyzing the data.
Methodology
The process of factor analysis involves several steps. First, the researcher collects and preprocesses the data, ensuring that it meets the assumptions of factor analysis, such as linearity, normality, and homoscedasticity. Next, a correlation matrix is computed to understand the relationships between variables. The extraction of factors is then performed using techniques like Principal Component Analysis (PCA) or Maximum Likelihood Estimation (MLE). After extraction, the factors are rotated to achieve a simpler and more interpretable structure, using methods like Varimax or Promax rotation. Finally, the results are interpreted, and the factors are given meaningful names based on the variables that load highly on them.
Applications
Factor analysis has a wide range of applications across various fields. In psychology and social sciences, it is used to identify underlying constructs such as personality traits, attitudes, and intelligence factors. In marketing, it helps in market segmentation and understanding consumer preferences. In finance, it is used to identify underlying risk factors that affect asset prices. Additionally, factor analysis is employed in the development of questionnaires and surveys, ensuring that they measure the intended constructs accurately.
Advantages and Limitations
One of the primary advantages of factor analysis is its ability to reduce the dimensionality of data, making it easier to interpret and analyze. It also helps in identifying latent variables that are not directly observable. However, factor analysis has its limitations. It requires large sample sizes to produce reliable results, and the interpretation of factors can be subjective. Moreover, the method assumes that the underlying relationships between variables are linear, which may not always be the case.
Conclusion
Factor analysis is a powerful statistical tool that aids in the simplification and interpretation of complex data sets. By identifying underlying factors, it provides valuable insights that can inform theory development, research, and practical applications across various domains. Despite its limitations, when used appropriately, factor analysis can significantly enhance our understanding of the data and the phenomena being studied.