Data Warehousing
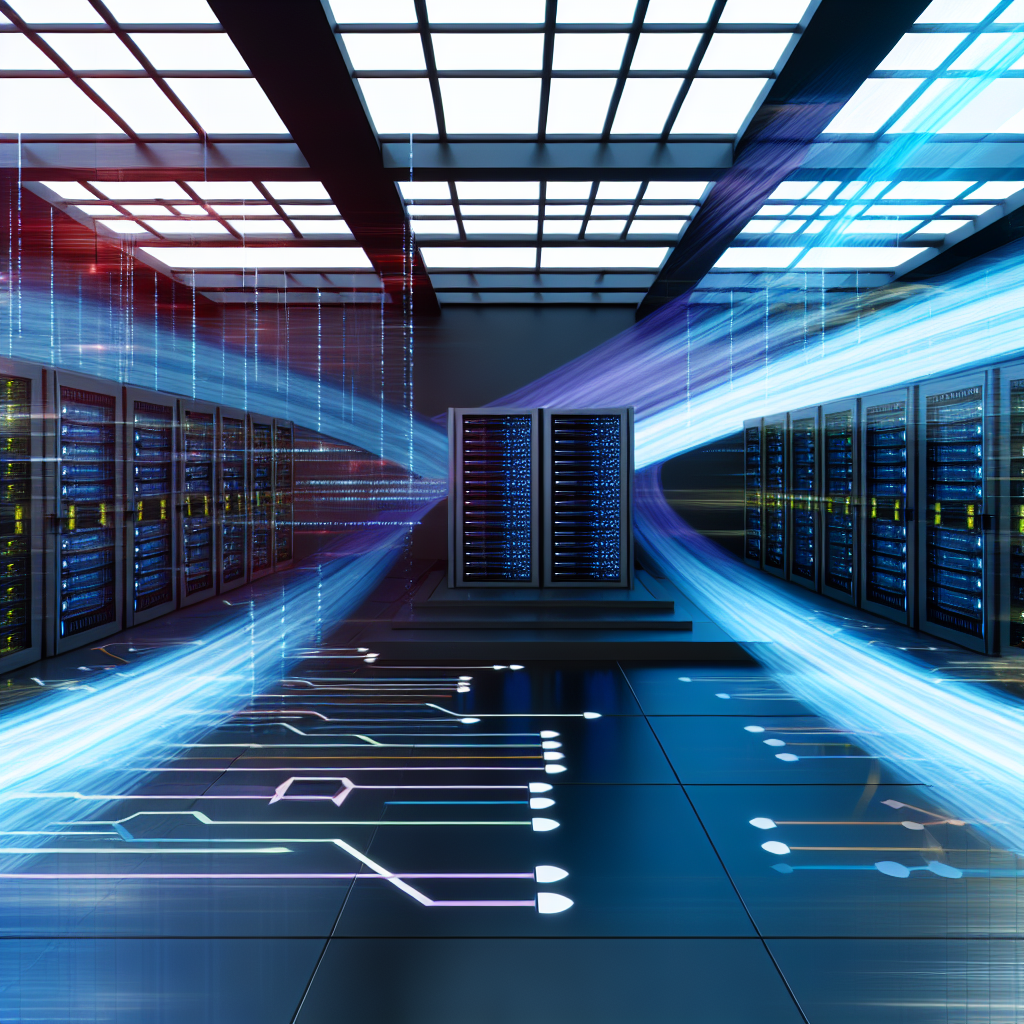
Table of Contents
Introduction
Data warehousing is a critical component in the realm of data management and business intelligence. It involves the collection, storage, and management of large volumes of data from various sources, enabling organizations to make informed decisions based on comprehensive data analysis. This article provides a detailed exploration of data warehousing, discussing its importance, architecture, benefits, and future trends.
In today’s data-driven world, businesses are inundated with vast amounts of data from multiple sources including transactional systems, social media, and IoT devices. Managing and analyzing this data to extract valuable insights poses a significant challenge. This is where data warehousing comes into play, offering a systematic approach to store and manage data efficiently.
Importance of Data Warehousing
Data warehousing plays a vital role in consolidating data from disparate sources into a single, unified repository. This consolidation facilitates easier access to data for analysis and reporting purposes. By centralizing data, organizations can ensure consistency, improve data quality, and enhance decision-making processes. Furthermore, data warehousing enables historical analysis, allowing businesses to identify trends and patterns over time.
Architecture of a Data Warehouse
The architecture of a data warehouse typically includes several key components. The first component is the data source layer, which comprises various data sources such as databases, flat files, and external data streams. The next component is the ETL (Extract, Transform, Load) process, which extracts data from source systems, transforms it into a suitable format, and loads it into the data warehouse. The data storage layer is where the transformed data is stored, often in a relational database optimized for read-heavy operations. Finally, the presentation layer involves tools and applications that enable users to query and analyze the data.
A well-designed data warehouse architecture ensures scalability, reliability, and performance. It is crucial to consider factors such as data volume, query complexity, and user concurrency when designing the architecture. Modern data warehouses often leverage cloud-based solutions, providing flexibility and scalability to handle growing data demands.
Benefits of Data Warehousing
Data warehousing offers numerous benefits to organizations. One of the primary advantages is improved decision-making. By providing a centralized repository of high-quality data, decision-makers can access accurate and timely information, leading to better business outcomes. Additionally, data warehousing enhances data consistency and integrity, as data from different sources is standardized and cleansed during the ETL process.
Another significant benefit is the ability to perform complex queries and analysis. Data warehouses are optimized for read-heavy operations, enabling users to run complex queries without impacting the performance of transactional systems. This capability is particularly valuable for business intelligence and reporting purposes. Moreover, data warehousing supports historical analysis, allowing organizations to track changes over time and gain deeper insights into business trends.
Future Trends in Data Warehousing
The field of data warehousing continues to evolve, driven by advancements in technology and changing business needs. One notable trend is the adoption of cloud-based data warehousing solutions. Cloud platforms offer scalability, flexibility, and cost-efficiency, making them an attractive option for organizations of all sizes. With cloud-based solutions, businesses can easily scale their data warehousing infrastructure to accommodate growing data volumes and changing requirements.
Another emerging trend is the integration of artificial intelligence (AI) and machine learning (ML) into data warehousing processes. AI and ML can enhance data processing, automate data cleansing, and provide predictive analytics capabilities. These technologies enable organizations to extract deeper insights from their data and make data-driven decisions with greater accuracy.
Additionally, real-time data warehousing is gaining traction. Traditional data warehouses often operate in batch mode, with data being updated periodically. Real-time data warehousing, on the other hand, allows for continuous data updates, enabling organizations to access the most current information for analysis and decision-making. This capability is particularly valuable in industries where timely data is critical, such as finance and healthcare.
Conclusion
In conclusion, data warehousing is an essential aspect of modern data management, providing organizations with the tools and infrastructure needed to store, manage, and analyze large volumes of data effectively. By centralizing data from various sources, data warehousing enhances decision-making, improves data quality, and supports complex queries and historical analysis. As technology continues to advance, data warehousing will evolve, incorporating cloud solutions, AI, and real-time capabilities to meet the growing demands of businesses. Organizations that invest in robust data warehousing solutions will be well-positioned to leverage their data for strategic advantage and drive business success.