Zero-shot Learning: The Future of AI and Machine Learning
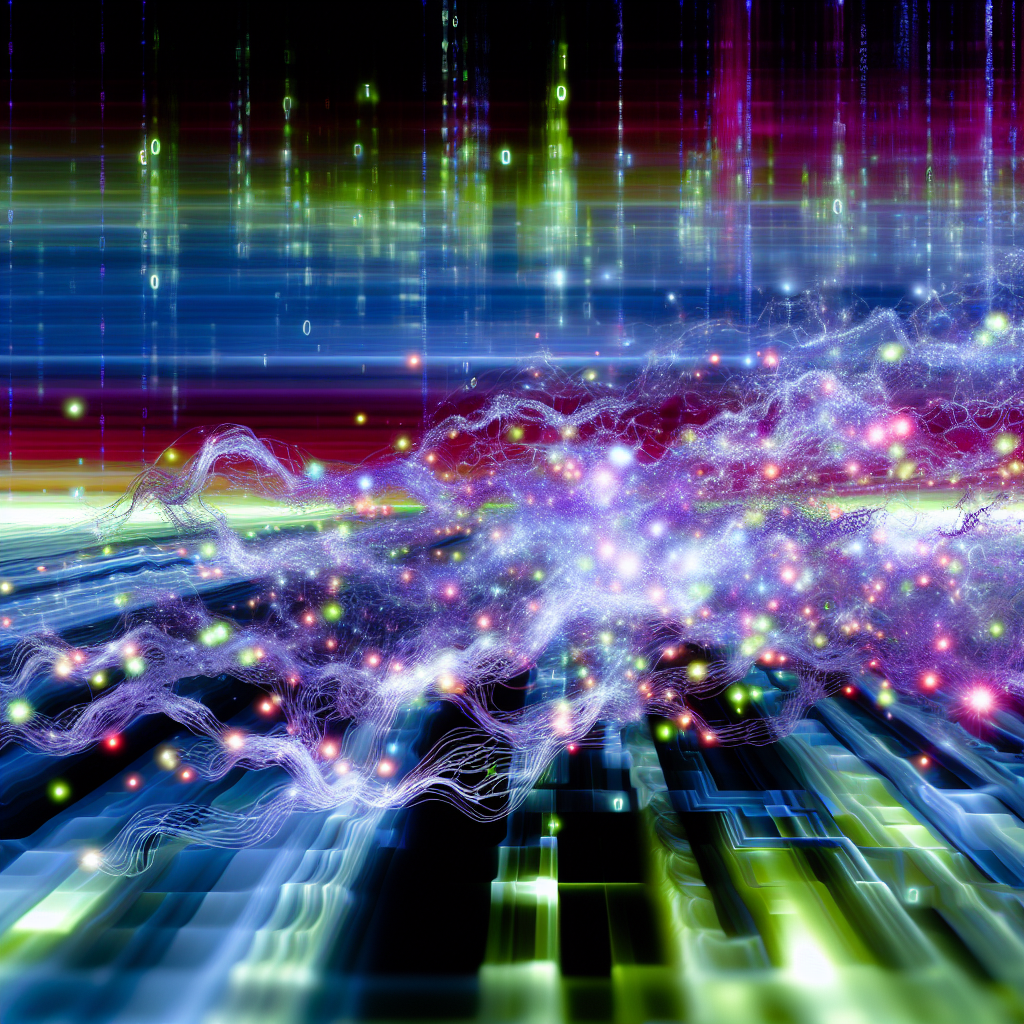
Table of Contents
Introduction to Zero-shot Learning
Zero-shot learning (ZSL) is a cutting-edge technique in the fields of artificial intelligence (AI) and machine learning. It refers to the ability of a model to correctly make predictions on tasks it has never encountered during its training phase. This remarkable capability is akin to a human being able to recognize or understand something entirely new based on prior knowledge and reasoning. Zero-shot learning is transforming the landscape of AI by enabling models to generalize their learning experiences to new, unseen situations without requiring additional data.
How Zero-shot Learning Works
Zero-shot learning operates on the principle of transferring knowledge from known tasks to unknown tasks. This is achieved through a combination of semantic embedding and attribute-based learning. Semantic embedding involves representing data in a high-dimensional space where similar items are closer together. For example, words or images can be embedded in a vector space where their relationships can be understood based on their distances and directions from each other. Attribute-based learning, on the other hand, involves defining a set of attributes or features that can be used to describe both known and unknown classes. By leveraging these attributes, a zero-shot learning model can infer the characteristics of new classes and make accurate predictions.
Applications of Zero-shot Learning
The applications of zero-shot learning are vast and varied, spanning numerous industries and domains. One of the most prominent applications is in image recognition, where ZSL models can identify objects or scenes that were not part of their training dataset. This is particularly useful in fields such as medical imaging, where new diseases or anomalies can be detected without the need for extensive labeled data. In natural language processing, zero-shot learning enables models to understand and translate languages they have never encountered before. This has significant implications for global communication and accessibility. Additionally, zero-shot learning is being used in recommendation systems, allowing for personalized suggestions based on user preferences and behaviors without requiring exhaustive data collection.
Challenges and Limitations
Despite its promising potential, zero-shot learning is not without its challenges and limitations. One of the primary challenges is the need for a robust and comprehensive set of attributes or features that can accurately describe both known and unknown classes. If the attributes are not well-defined or insufficient, the model’s predictions may be inaccurate or unreliable. Another limitation is the reliance on high-quality semantic embeddings. Poorly constructed embeddings can lead to incorrect relationships and associations, thereby affecting the model’s performance. Furthermore, zero-shot learning models can be computationally intensive, requiring significant processing power and memory to handle the complex calculations involved.
Future Prospects of Zero-shot Learning
The future prospects of zero-shot learning are incredibly promising, with ongoing research and advancements poised to overcome current challenges and expand its capabilities. The integration of zero-shot learning with other AI techniques, such as reinforcement learning and generative models, holds potential for even more sophisticated and adaptable systems. As computational power continues to grow and data becomes increasingly accessible, zero-shot learning models are expected to become more efficient and effective. The ability to generalize learning to new and unseen scenarios will be a cornerstone of future AI systems, enabling them to operate in dynamic and unpredictable environments with minimal human intervention.
Conclusion
In conclusion, zero-shot learning represents a significant leap forward in the field of artificial intelligence and machine learning. By enabling models to make accurate predictions without prior exposure to specific tasks, ZSL is paving the way for more versatile and intelligent systems. While there are still challenges to be addressed, the potential applications and benefits of zero-shot learning are immense. As research and technology continue to advance, zero-shot learning will undoubtedly play a crucial role in shaping the future of AI, making it more capable, adaptable, and accessible to a wider range of industries and applications.