Swarm Intelligence: Harnessing the Power of Collective Behavior
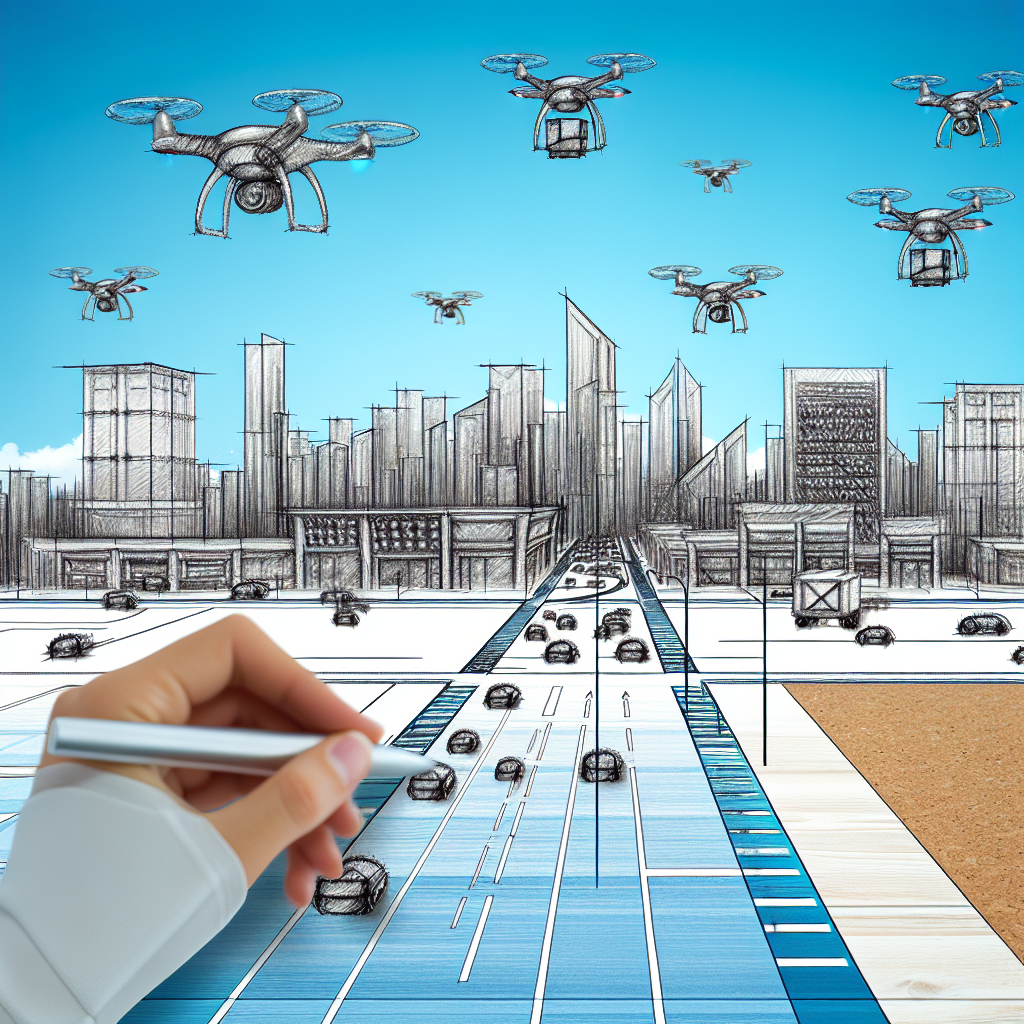
Table of Contents
Introduction to Swarm Intelligence
Swarm intelligence is a fascinating concept that has gained significant attention in the fields of artificial intelligence, robotics, and computational science. It refers to the collective behavior of decentralized, self-organized systems, typically composed of simple agents that interact locally with one another and their environment. The inspiration for swarm intelligence comes from nature, where we can observe complex behaviors in groups of animals, such as flocks of birds, schools of fish, and colonies of ants.
Historical Background
The term ‘swarm intelligence’ was first introduced by Gerardo Beni and Jing Wang in 1989, in the context of cellular robotic systems. However, the principles behind this concept have been studied for centuries. Early observations of animal behavior laid the groundwork for understanding how individual actions can lead to emergent, intelligent group behavior. Over the years, researchers have developed algorithms and models that mimic these natural systems to solve complex problems in various domains.
Key Principles
Swarm intelligence relies on several key principles: decentralization, self-organization, and simple rules. In a decentralized system, there is no central authority dictating the actions of each agent. Instead, each agent follows simple rules based on local information and interactions. This self-organization leads to the emergence of complex, adaptive behaviors that are often more efficient and resilient than those engineered through centralized control.
Applications in Technology
Swarm intelligence has a wide range of applications in technology. One of the most well-known applications is in robotics, where groups of robots, or ‘swarms’, can perform tasks collectively that would be difficult or impossible for a single robot to achieve. For example, swarm robotics can be used for search and rescue missions, environmental monitoring, and agricultural tasks. Additionally, swarm intelligence algorithms are used in optimization problems, such as the traveling salesman problem, network routing, and load balancing.
Natural Inspirations
Many swarm intelligence algorithms are inspired by natural systems. For instance, ant colony optimization (ACO) is based on the foraging behavior of ants, which find the shortest path to food sources by laying down pheromones. Particle swarm optimization (PSO) is inspired by the social behavior of birds flocking or fish schooling. These natural inspirations provide valuable insights into designing efficient and robust algorithms for complex problem-solving.
Challenges and Limitations
Despite its potential, swarm intelligence is not without challenges and limitations. One of the main challenges is ensuring reliable communication and coordination among agents, especially in dynamic and unpredictable environments. Additionally, designing simple rules that lead to desired emergent behaviors can be a complex task. There are also scalability issues, as the number of agents increases, the computational and communication overhead can become significant.
Future Directions
The future of swarm intelligence holds exciting possibilities. Advances in artificial intelligence, machine learning, and sensor technologies are likely to enhance the capabilities of swarm systems. Researchers are exploring hybrid approaches that combine swarm intelligence with other computational techniques to improve performance and scalability. Moreover, there is growing interest in applying swarm intelligence to new areas, such as healthcare, urban planning, and autonomous transportation systems.
Conclusion
Swarm intelligence represents a powerful paradigm for solving complex problems through collective behavior. By mimicking natural systems, researchers and engineers can develop robust, adaptive solutions that leverage the strengths of decentralized, self-organized systems. As technology continues to evolve, the potential applications of swarm intelligence will likely expand, offering innovative solutions to some of the most pressing challenges in various fields.