Sentiment Analysis: Unveiling the Emotions in Text
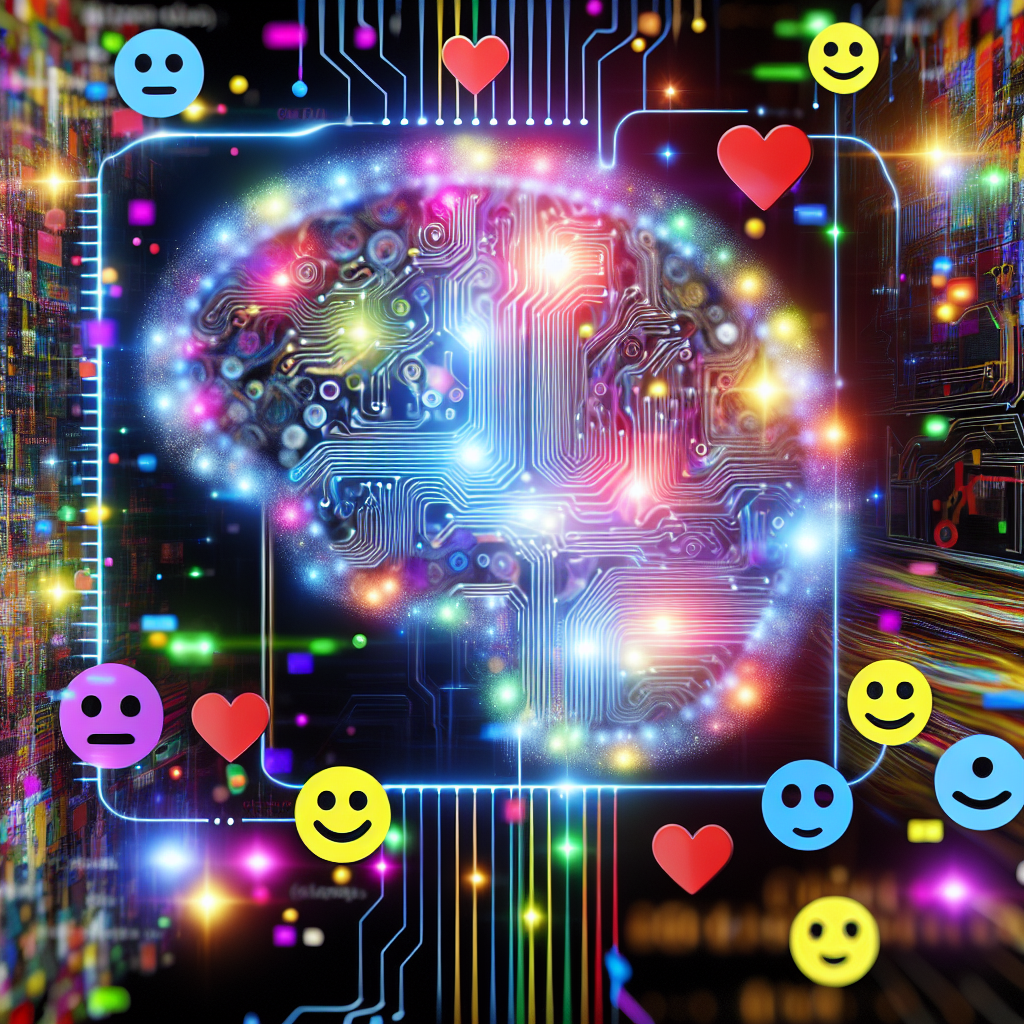
Table of Contents
Introduction
Sentiment analysis, also known as opinion mining, is a powerful tool in the realm of natural language processing (NLP). It involves the use of algorithms and machine learning to identify and extract subjective information from various textual data sources. The primary goal is to determine the sentiment expressed in a piece of text, which can be positive, negative, or neutral. This technique has gained immense popularity due to its applications in diverse fields such as marketing, customer service, finance, and more.
The significance of sentiment analysis lies in its ability to transform raw text data into structured information that can be used to make informed decisions. By understanding the emotions and opinions of customers, businesses can tailor their strategies to better meet the needs and expectations of their target audience. Moreover, sentiment analysis can also be used to monitor brand reputation, gauge public opinion on various topics, and even predict market trends.
How Sentiment Analysis Works
Sentiment analysis typically involves several steps, starting with the collection of textual data from sources such as social media platforms, customer reviews, or survey responses. This data is then preprocessed to remove any noise, such as irrelevant information or special characters. Next, the text is tokenized, meaning it is broken down into smaller units like words or phrases. These tokens are then analyzed using various techniques to determine their sentiment.
One common approach to sentiment analysis is the use of lexicons, which are pre-defined lists of words associated with positive or negative sentiments. For instance, words like ’excellent’ and ‘happy’ might be considered positive, while words like ’terrible’ and ‘sad’ might be considered negative. By comparing the tokens in the text to the words in the lexicon, the algorithm can assign a sentiment score to the entire text. However, this method has its limitations, as it may not account for context or the nuances of language.
Advanced Techniques in Sentiment Analysis
More advanced techniques in sentiment analysis involve the use of machine learning models, which can be trained on large datasets to recognize patterns and make predictions. These models can be supervised, unsupervised, or semi-supervised, depending on whether they are trained with labeled data or not. Supervised learning involves training the model on a dataset where the sentiment of each text is already known, allowing the model to learn from these examples and apply its knowledge to new, unseen data.
Unsupervised learning, on the other hand, does not rely on labeled data. Instead, the model identifies patterns and relationships within the data on its own. This approach can be useful when labeled data is scarce or unavailable. Semi-supervised learning combines elements of both, using a small amount of labeled data to guide the learning process while allowing the model to explore and learn from the larger, unlabeled dataset.
Applications of Sentiment Analysis
Sentiment analysis has a wide range of applications across various industries. In marketing, it can be used to analyze customer feedback and social media mentions to understand how consumers feel about a brand or product. This information can then be used to develop targeted marketing campaigns, improve customer service, and enhance product offerings. In finance, sentiment analysis can help predict stock market movements by analyzing news articles, financial reports, and social media posts for market sentiment.
The healthcare industry can also benefit from sentiment analysis by monitoring patient feedback and identifying areas for improvement in patient care. Additionally, sentiment analysis can be used in politics to gauge public opinion on policies and candidates, helping to shape campaign strategies and policy decisions. Overall, the ability to understand and interpret human emotions through text has far-reaching implications and benefits.
Challenges and Future Directions
Despite its many advantages, sentiment analysis is not without its challenges. One of the main difficulties is dealing with the complexity and ambiguity of human language. Sarcasm, irony, and context can all affect the sentiment of a text, making it difficult for algorithms to accurately interpret the intended meaning. Additionally, the ever-evolving nature of language means that sentiment analysis models need to be continuously updated and refined to remain effective.
Looking to the future, advancements in NLP and machine learning are expected to further enhance the capabilities of sentiment analysis. Techniques such as deep learning and transformer models, like BERT and GPT-3, are already showing promise in improving the accuracy and efficiency of sentiment analysis. As these technologies continue to evolve, we can expect sentiment analysis to become an even more integral part of data-driven decision-making processes across various domains.
Conclusion
In conclusion, sentiment analysis is a powerful tool that enables the extraction of valuable insights from textual data. By understanding the emotions and opinions expressed in text, businesses and individuals can make more informed decisions and better connect with their audiences. While there are challenges to overcome, ongoing advancements in technology are paving the way for more accurate and effective sentiment analysis techniques. As we continue to explore and refine these methods, the potential applications and benefits of sentiment analysis will only continue to grow.