Recommendation Systems
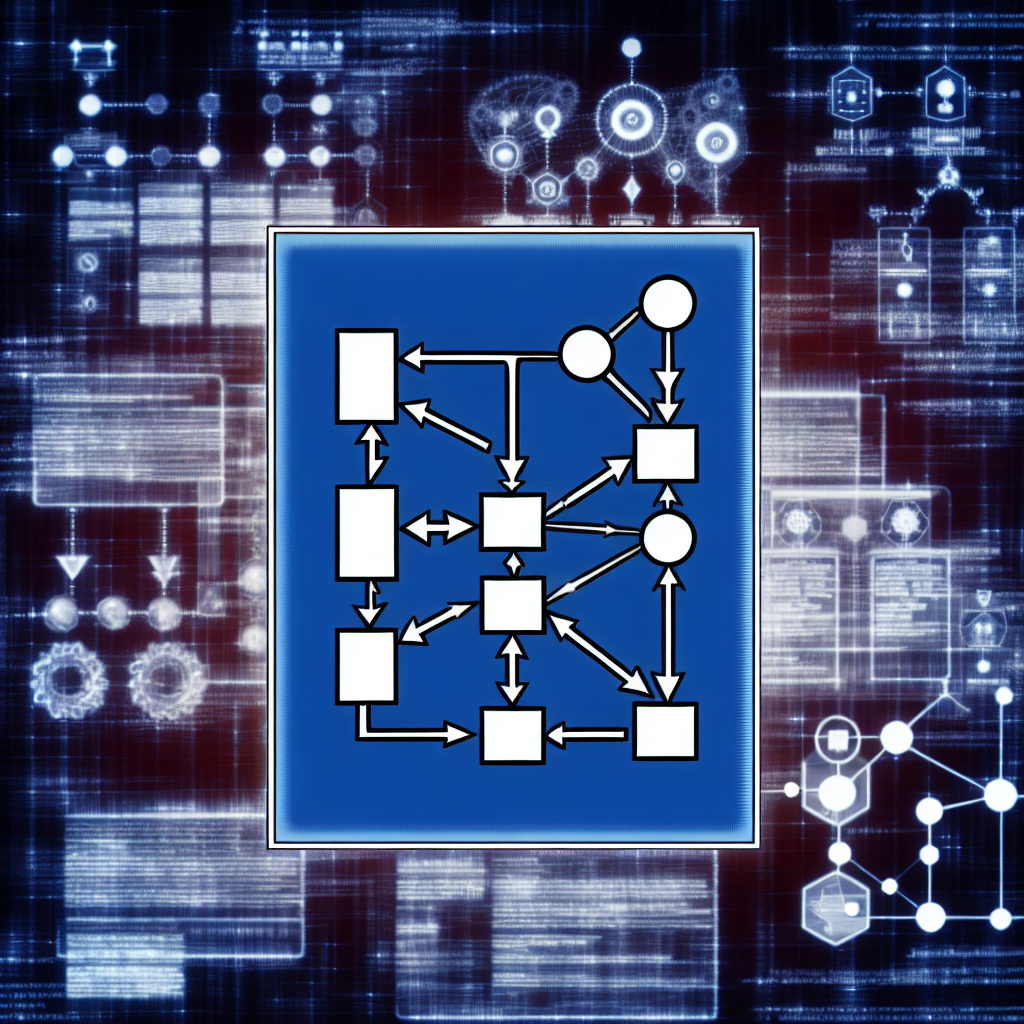
Table of Contents
Introduction
Recommendation systems have become an integral part of our daily digital interactions. Whether we are shopping online, streaming movies, or browsing social media, these systems are working behind the scenes to provide us with personalized suggestions. This article delves into the intricacies of recommendation systems, exploring their types, mechanisms, and applications across various industries.
What are Recommendation Systems?
Recommendation systems, also known as recommender systems, are algorithms designed to suggest relevant items to users. These items could be products, movies, books, articles, or any other form of content. The primary goal of these systems is to enhance user experience by offering personalized recommendations based on user preferences and behavior.
Types of Recommendation Systems
Broadly speaking, recommendation systems can be classified into three main types: collaborative filtering, content-based filtering, and hybrid models. Each type has its unique approach and advantages.
Collaborative Filtering
Collaborative filtering is one of the most popular methods used in recommendation systems. It relies on the collective preferences of many users to recommend items. The idea is that if users A and B have similar tastes, the items liked by user A can be recommended to user B. There are two main types of collaborative filtering: user-based and item-based. User-based collaborative filtering finds users who are similar to the target user and recommends items that those similar users have liked. Item-based collaborative filtering, on the other hand, recommends items that are similar to items the target user has liked in the past.
Content-Based Filtering
Content-based filtering focuses on the attributes of the items themselves rather than user interactions. It recommends items that are similar to those the user has shown interest in. For instance, if a user has watched and liked several action movies, the system will recommend other action movies based on their genre, director, actors, and other attributes. This method requires a deep understanding of the content’s features and how they relate to user preferences.
Hybrid Models
Hybrid recommendation systems combine the strengths of both collaborative filtering and content-based filtering to provide more accurate and effective recommendations. By leveraging multiple data sources and algorithms, hybrid models can overcome the limitations of individual methods. For example, they can address the cold start problem, where new users or items with little interaction data may not receive accurate recommendations. Hybrid systems can use content-based filtering to recommend items initially and then switch to collaborative filtering as more user interaction data becomes available.
Applications of Recommendation Systems
Recommendation systems have a wide range of applications across various industries. In e-commerce, they help increase sales by suggesting products that customers are likely to buy. In the entertainment industry, they enhance user experience by recommending movies, music, and TV shows tailored to individual tastes. Social media platforms use recommendation systems to personalize content feeds, connecting users with relevant posts, friends, and groups. Additionally, news websites use these systems to suggest articles that match readers’ interests, thereby increasing engagement and retention.
Challenges and Future Directions
Despite their widespread use and success, recommendation systems face several challenges. One major issue is the cold start problem, where new users or items lack sufficient interaction data for accurate recommendations. Another challenge is the scalability of algorithms to handle large datasets efficiently. Privacy concerns also arise as these systems collect and analyze vast amounts of user data. Looking ahead, the future of recommendation systems lies in addressing these challenges through advancements in machine learning, artificial intelligence, and data privacy technologies. Continuous research and development are essential to enhance the accuracy, efficiency, and ethical aspects of these systems.
Conclusion
Recommendation systems play a crucial role in shaping our digital experiences by providing personalized suggestions. From collaborative filtering and content-based filtering to hybrid models, these systems utilize various techniques to understand and predict user preferences. As technology continues to evolve, recommendation systems will become even more sophisticated, offering more accurate and relevant recommendations while addressing challenges related to data privacy and scalability. The ongoing advancements in this field promise a future where our interactions with digital content are more intuitive and personalized than ever before.