Predictive Modeling
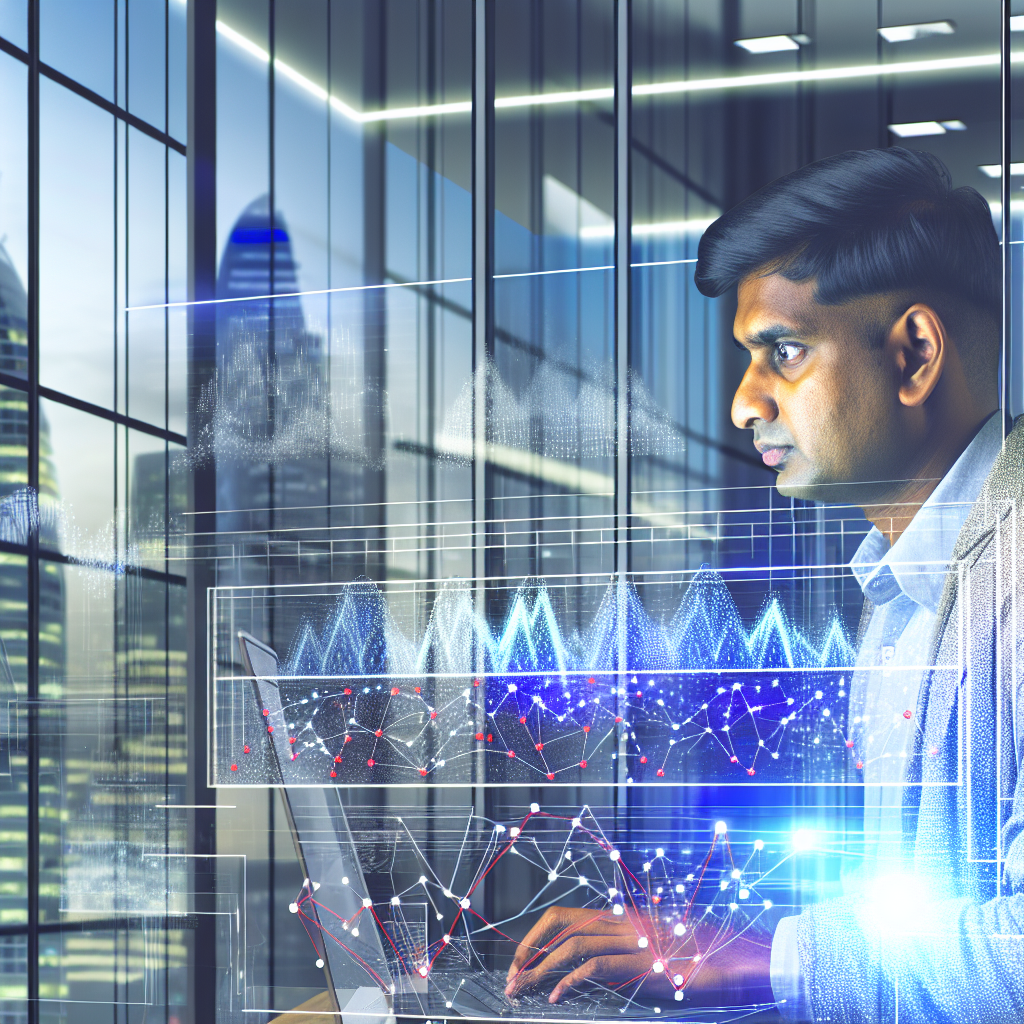
Table of Contents
Predictive modeling is a powerful statistical technique that leverages data mining and probability to forecast outcomes. By analyzing current and historical data, predictive models can provide insights into future events, helping organizations make informed decisions. This method is widely used in various fields such as finance, healthcare, marketing, and more.
## The Basics of Predictive Modeling
At its core, predictive modeling involves the use of mathematical algorithms and statistical techniques to identify patterns within a dataset. These patterns are then used to predict future occurrences. The process typically starts with data collection and preprocessing, followed by model selection, training, validation, and finally, deployment. Each step is crucial for ensuring the accuracy and reliability of the predictions.
### Data Collection and Preprocessing
Data collection is the foundation of predictive modeling. It involves gathering relevant data from various sources such as databases, APIs, or direct input from users. Once collected, the data needs to be cleaned and preprocessed to remove any inconsistencies or errors. This step may involve handling missing values, normalizing data, and transforming variables to suitable formats for analysis.
### Model Selection and Training
Selecting the right model is a critical step in predictive modeling. There are various algorithms to choose from, including linear regression, decision trees, neural networks, and more. Each algorithm has its strengths and weaknesses, and the choice depends on the specific requirements of the problem at hand. Once a model is selected, it is trained using a subset of the data. During training, the model learns to recognize patterns and relationships within the data.
### Validation and Deployment
After training, the model must be validated to ensure its effectiveness. This involves testing the model on a separate dataset to evaluate its performance. Common validation techniques include cross-validation, holdout validation, and bootstrapping. Once validated, the model can be deployed in a real-world environment to make predictions. Continuous monitoring and updating of the model are essential to maintain its accuracy over time.
## Applications of Predictive Modeling
Predictive modeling has a wide range of applications across various industries. In finance, it is used for credit scoring, fraud detection, and risk management. Healthcare professionals use predictive models to forecast patient outcomes, optimize treatment plans, and manage resources. In marketing, predictive modeling helps businesses understand customer behavior, personalize marketing campaigns, and improve customer retention. The possibilities are virtually endless, and the impact of predictive modeling continues to grow as more data becomes available.
## The Future of Predictive Modeling
The future of predictive modeling looks promising, with advancements in artificial intelligence and machine learning driving the field forward. As computational power increases and data collection methods improve, predictive models will become more sophisticated and accurate. Additionally, the integration of predictive modeling with other technologies such as the Internet of Things (IoT) and big data analytics will open up new opportunities for innovation and efficiency across various sectors.
In conclusion, predictive modeling is a transformative tool that enables organizations to harness the power of data for strategic decision-making. By understanding and anticipating future events, businesses can gain a competitive edge, optimize operations, and deliver better outcomes. As technology continues to evolve, the potential of predictive modeling will only expand, making it an indispensable asset in the modern world.