Machine Translation: Bridging Language Barriers with Technology
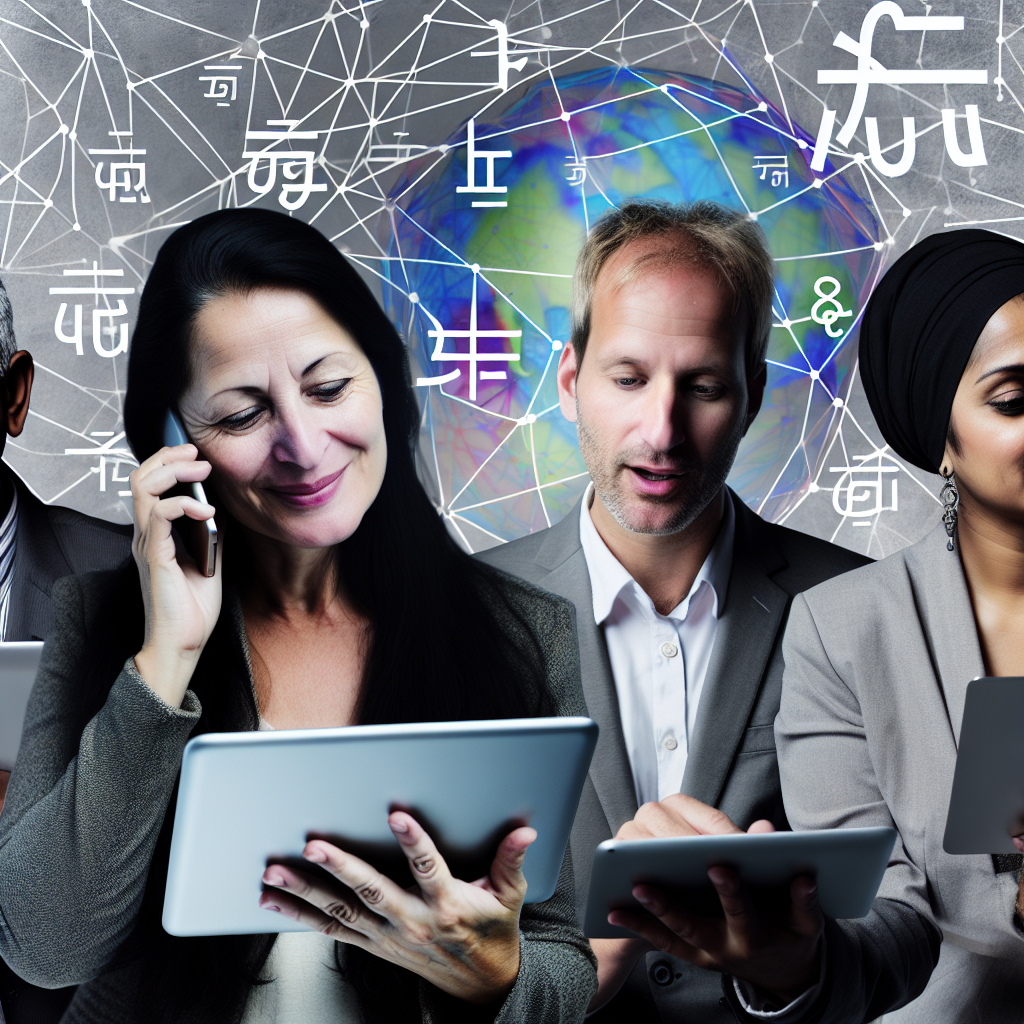
Table of Contents
Introduction to Machine Translation
Machine translation (MT) refers to the use of computer software to translate text or speech from one language to another. It is a branch of computational linguistics that has significantly evolved over the past few decades. The aim of MT is to break down language barriers and facilitate global communication, making information accessible to a wider audience regardless of linguistic differences. This technology is increasingly vital in our interconnected world, where cross-cultural communication is essential for business, education, and social interactions.
Historical Background
The concept of machine translation dates back to the 1950s, with early efforts focusing on rule-based approaches. These initial systems relied heavily on linguistic rules and dictionaries to perform translations. The first significant milestone was the Georgetown-IBM experiment in 1954, which successfully translated more than sixty Russian sentences into English. Despite its limited scope, this experiment demonstrated the potential of MT and spurred further research and development. Over the years, MT systems have evolved from rule-based methods to more advanced techniques like statistical machine translation (SMT) and, more recently, neural machine translation (NMT).
Evolution of Machine Translation Methods
Machine translation methods can be broadly categorized into three main types: rule-based, statistical, and neural. Rule-based machine translation (RBMT) relies on linguistic rules and bilingual dictionaries. It requires extensive manual work to create and maintain the rules, making it less scalable. Statistical machine translation (SMT) emerged in the late 1980s and early 1990s, leveraging large corpora of bilingual text to generate translations based on statistical models. SMT improved translation quality but often struggled with idiomatic expressions and context understanding. The most recent and advanced method is neural machine translation (NMT), which uses deep learning techniques and artificial neural networks to produce more accurate and fluent translations. NMT models, such as Google’s Transformer model, have set new standards in the field by understanding context and nuances better than previous methods.
Applications and Impact
Machine translation has a wide range of applications across various domains. In business, it enables companies to reach global markets by translating marketing materials, websites, and customer support content. In education, MT helps students and researchers access academic resources in different languages, fostering knowledge exchange. Social media platforms use MT to allow users to communicate across language barriers, promoting global interactions. The impact of MT is also evident in humanitarian efforts, where it aids in crisis communication and information dissemination in multilingual regions. Overall, MT enhances accessibility, efficiency, and inclusivity, making it a crucial tool in today’s digital age.
Challenges and Limitations
Despite significant advancements, machine translation still faces several challenges. One major issue is the quality of translations, especially for languages with less available data. NMT systems require large amounts of data to train effectively, which can be a limitation for less commonly spoken languages. Additionally, MT systems often struggle with context, idiomatic expressions, and cultural nuances, resulting in translations that may be grammatically correct but semantically inaccurate. Another challenge is the potential bias in translation models, which can perpetuate stereotypes and inaccuracies. Addressing these challenges requires ongoing research, improvements in data collection, and the development of more sophisticated models.
Future Directions
The future of machine translation holds exciting possibilities. Researchers are exploring ways to improve the accuracy and fluency of translations by integrating more advanced AI techniques and leveraging larger, more diverse datasets. Multimodal translation, which involves translating text in the context of images or videos, is an emerging area that promises to enhance the understanding of context and improve translation quality. Moreover, efforts are being made to create more inclusive translation systems that cater to a broader range of languages and dialects. As technology continues to advance, MT is expected to become even more integral to global communication, breaking down language barriers and fostering a more connected world.
In conclusion, machine translation is a transformative technology that has made significant strides in bridging language barriers. From its early rule-based systems to the sophisticated neural models of today, MT continues to evolve and improve. Its applications span various domains, enhancing global communication, accessibility, and inclusivity. While challenges remain, ongoing research and technological advancements hold promise for even more accurate and effective translation systems in the future. As we move towards an increasingly interconnected world, machine translation will undoubtedly play a crucial role in facilitating cross-cultural understanding and cooperation.