Explainable AI: Unveiling the Mysteries of Machine Learning
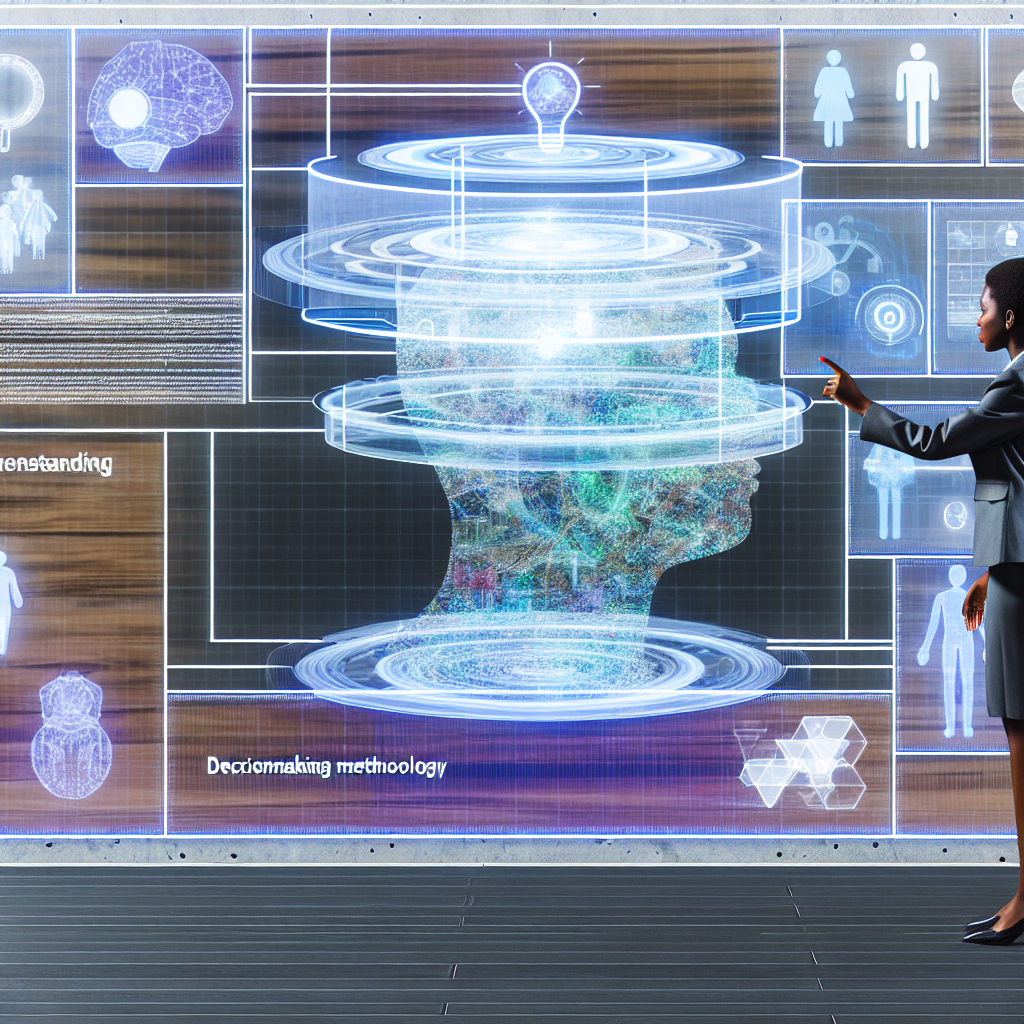
Table of Contents
Introduction
Artificial Intelligence (AI) and Machine Learning (ML) have become ubiquitous in today’s technological landscape. From healthcare to finance, AI-driven solutions are transforming industries by providing unprecedented insights and automating complex tasks. However, as these systems become more ingrained in our daily lives, the need for transparency and understanding of how they make decisions has become paramount. This is where Explainable AI (XAI) comes into play.
Explainable AI refers to techniques and methods that make the decision-making processes of AI systems transparent and understandable to humans. Unlike traditional AI models, which often operate as ‘black boxes,’ XAI aims to provide clear and interpretable explanations for the outcomes generated by these systems. This not only helps in building trust among users but also ensures that AI systems can be held accountable for their actions.
The Importance of Explainable AI
The significance of Explainable AI cannot be overstated. In critical fields such as healthcare, finance, and law enforcement, the decisions made by AI systems can have far-reaching consequences. For instance, in medical diagnostics, an AI model might predict the likelihood of a patient having a particular disease. If the rationale behind this prediction is not clear, it becomes challenging for healthcare professionals to trust and act upon the AI’s recommendation. Explainable AI helps in bridging this gap by elucidating the factors that influenced the model’s predictions.
Moreover, regulatory compliance is another compelling reason for adopting XAI. Governments and regulatory bodies are increasingly emphasizing the need for transparency in AI-driven decisions. For example, the European Union’s General Data Protection Regulation (GDPR) mandates that individuals have the right to an explanation for decisions made by automated systems. By implementing XAI, organizations can ensure compliance with such regulations and avoid potential legal repercussions.
Challenges in Implementing Explainable AI
Despite its benefits, the implementation of Explainable AI is fraught with challenges. One of the primary obstacles is the inherent complexity of modern AI models. Deep learning algorithms, which are widely used in AI applications, consist of numerous layers and parameters that interact in intricate ways. Simplifying these complex models without compromising their accuracy is a daunting task.
Another challenge lies in the trade-off between interpretability and performance. Often, more interpretable models, such as decision trees, may not perform as well as complex models like neural networks. Striking a balance between providing meaningful explanations and maintaining high performance is a key challenge for researchers and practitioners in the field of XAI.
Real-World Applications of Explainable AI
Despite these challenges, there are several real-world applications where Explainable AI is making a significant impact. In the financial sector, XAI is used to enhance the transparency of credit scoring models. By understanding the factors that influence credit decisions, both lenders and borrowers can gain better insights into the lending process, leading to fairer and more informed decisions.
In the realm of healthcare, Explainable AI is being employed to improve diagnostic accuracy and patient outcomes. For example, AI models used in radiology can highlight specific areas of medical images that contributed to a diagnosis, allowing radiologists to verify and trust the AI’s findings. This collaborative approach enhances the overall quality of care provided to patients.
Law enforcement agencies are also leveraging XAI to ensure that AI-driven surveillance and predictive policing tools are used responsibly. By providing clear explanations for the decisions made by these systems, agencies can avoid biases and ensure that their operations are transparent and accountable to the public.
Future Directions
The field of Explainable AI is rapidly evolving, with ongoing research aimed at developing more effective and user-friendly explanation methods. Future advancements may include the integration of natural language processing techniques to generate explanations in human-readable formats, as well as the development of standardized metrics for evaluating the quality of explanations.
Furthermore, interdisciplinary collaboration between AI researchers, domain experts, and ethicists will be crucial in addressing the ethical and societal implications of XAI. By fostering a holistic approach, the AI community can ensure that the benefits of Explainable AI are realized while mitigating potential risks.
Conclusion
In conclusion, Explainable AI represents a vital step towards making AI systems more transparent, trustworthy, and accountable. As AI continues to permeate various aspects of our lives, the ability to understand and explain its decisions will be essential in building public trust and ensuring ethical use. While challenges remain, the ongoing advancements in XAI hold great promise for a future where AI systems are not only powerful but also comprehensible and fair.