Deep Learning
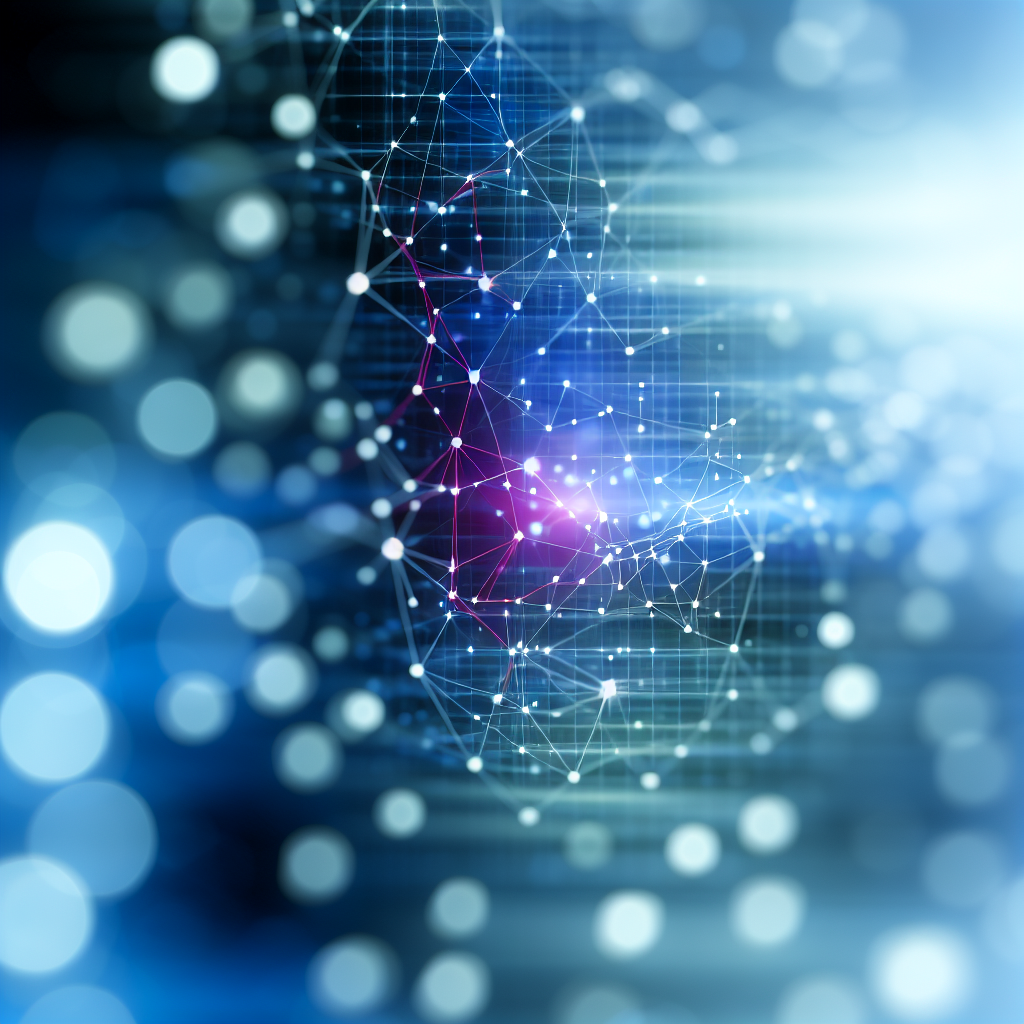
Table of Contents
Introduction
Deep learning is a subset of machine learning in artificial intelligence (AI) that has garnered significant attention in recent years. It involves the use of neural networks with many layers, or ‘deep’ structures, to analyze various forms of data. This technology has revolutionized fields such as computer vision, natural language processing, and speech recognition, among others. As we delve deeper into this topic, we will explore its origins, key concepts, applications, and future potential.
Historical Background
The journey of deep learning began in the mid-20th century with the advent of artificial neural networks (ANNs). The concept of a neural network was inspired by the human brain’s structure, consisting of neurons connected by synapses. Early pioneers like Frank Rosenblatt, who developed the Perceptron in 1957, laid the groundwork for future advancements. However, it wasn’t until the 1980s and 1990s that significant progress was made, thanks to the development of backpropagation algorithms. These algorithms enabled more efficient training of neural networks, leading to better performance and broader applications.
Core Concepts
At its core, deep learning involves the use of multi-layered neural networks to model complex patterns in data. Each layer in a neural network consists of nodes, or ’neurons’, which process input data and pass it on to the next layer. The depth of the network allows it to capture intricate patterns and relationships in the data. Key concepts in deep learning include activation functions, loss functions, and optimization algorithms. Activation functions, such as ReLU and sigmoid, introduce non-linearity into the model, enabling it to learn more complex patterns. Loss functions measure the difference between the predicted and actual outcomes, guiding the optimization process to minimize errors. Optimization algorithms, like stochastic gradient descent (SGD), adjust the model’s parameters to improve its performance.
Applications
Deep learning has a wide range of applications across various industries. In computer vision, it powers technologies like image recognition, object detection, and facial recognition. Deep learning models can identify and classify objects in images with remarkable accuracy, making them invaluable in fields such as healthcare, where they assist in diagnosing diseases from medical images. In natural language processing (NLP), deep learning enables machines to understand and generate human language. This has led to advancements in machine translation, sentiment analysis, and chatbots. Speech recognition systems, like those used in virtual assistants, also rely heavily on deep learning to convert spoken language into text and respond appropriately.
Challenges and Limitations
Despite its successes, deep learning faces several challenges and limitations. One of the primary challenges is the need for large amounts of labeled data for training. Collecting and annotating such data can be time-consuming and expensive. Additionally, deep learning models are computationally intensive, requiring significant processing power and memory. This often necessitates the use of specialized hardware, such as GPUs and TPUs. Another limitation is the ‘black box’ nature of deep learning models, which makes it difficult to interpret their decision-making processes. This lack of transparency can be problematic in applications where explainability is crucial, such as in healthcare and finance.
Future Prospects
The future of deep learning holds immense potential. Researchers are continually developing new techniques and architectures to overcome current limitations and enhance performance. One promising area of research is unsupervised and semi-supervised learning, which aims to reduce the reliance on labeled data. Another exciting development is the integration of deep learning with other AI technologies, such as reinforcement learning and transfer learning. These approaches can enable more efficient learning and adaptation to new tasks. As computational resources continue to improve, we can expect deep learning to drive further advancements in AI, leading to more intelligent and autonomous systems.
In conclusion, deep learning has emerged as a powerful tool in the realm of artificial intelligence. Its ability to model complex patterns and relationships in data has led to groundbreaking applications across various domains. While challenges remain, ongoing research and technological advancements promise to unlock even greater potential in the future. As we continue to explore and harness the capabilities of deep learning, it will undoubtedly play a pivotal role in shaping the future of AI and technology.