AI for Sentiment Prediction
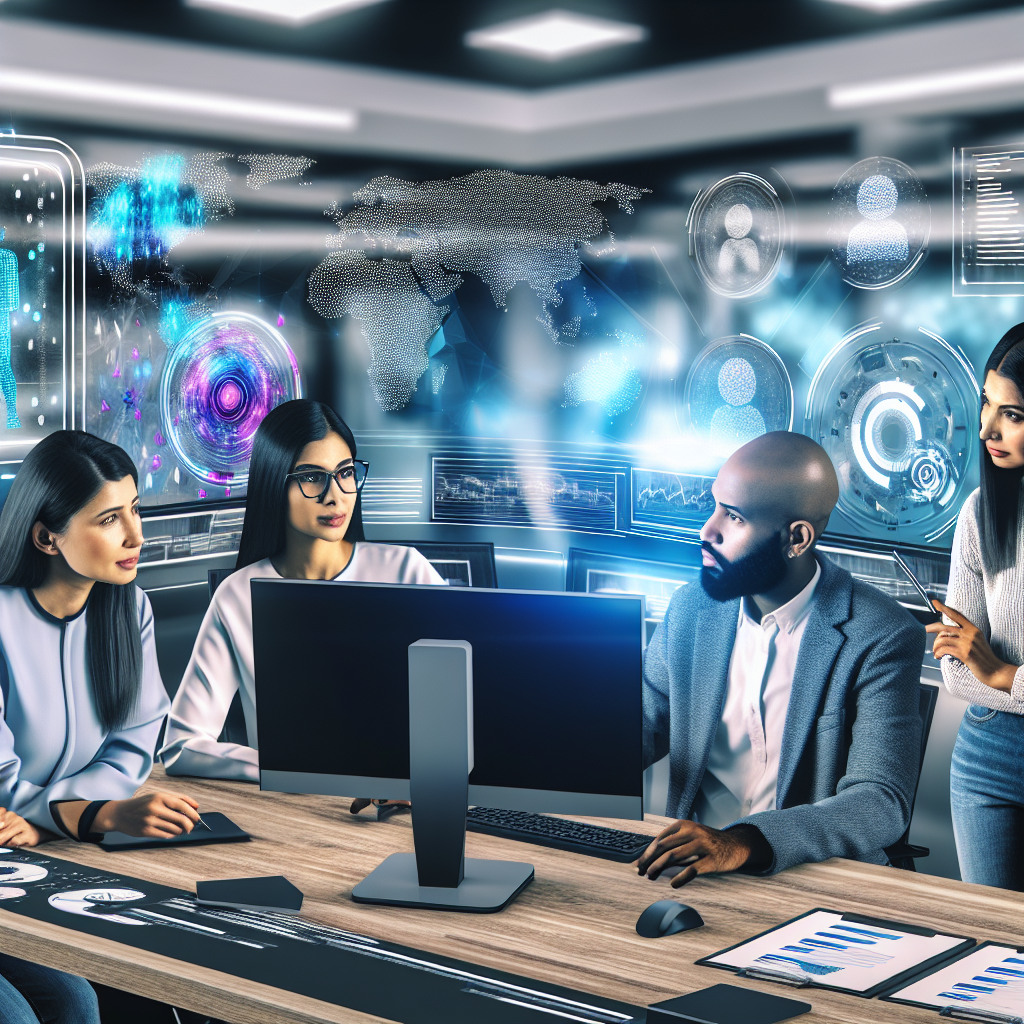
Table of Contents
Introduction
The advent of artificial intelligence (AI) has brought about a myriad of advancements across various fields, one of which is sentiment prediction. This technology leverages AI to determine the underlying emotions expressed in textual data. Whether it’s analyzing customer reviews, social media posts, or any other form of written communication, sentiment prediction offers invaluable insights that can drive decision-making processes in businesses, politics, and beyond.
The Mechanics of Sentiment Prediction
Sentiment prediction, also known as sentiment analysis, is primarily powered by natural language processing (NLP) and machine learning algorithms. NLP allows computers to understand, interpret, and respond to human language in a valuable way. Machine learning, on the other hand, involves training models on vast datasets to recognize patterns and make predictions. By combining these two technologies, AI systems can analyze text and predict whether the sentiment is positive, negative, or neutral.
Applications in Various Industries
The applications of AI for sentiment prediction are vast and varied. In the business sector, companies use sentiment analysis to gauge customer satisfaction and improve their products or services. For instance, by analyzing customer feedback on social media or review sites, businesses can quickly identify and address issues. In the political arena, sentiment prediction can analyze public opinion on policies or candidates, providing valuable insights for campaign strategies. Additionally, in the financial industry, sentiment analysis can predict stock market trends based on news articles and social media sentiment.
Challenges and Limitations
Despite its numerous benefits, AI for sentiment prediction is not without challenges. One major issue is the complexity of human language. Sarcasm, slang, and cultural nuances can often lead to misinterpretations. Furthermore, the quality of the training data significantly impacts the model’s accuracy. Bias in the data can lead to skewed results, which is particularly concerning in sensitive areas such as hiring or lending decisions. Ensuring diversity and inclusivity in training datasets is crucial to mitigate these biases.
Future Prospects
The future of AI for sentiment prediction looks promising, with continuous advancements in technology and methodologies. Enhanced NLP techniques and more sophisticated machine learning models are expected to improve the accuracy and reliability of sentiment analysis. Additionally, as more diverse and comprehensive datasets become available, AI systems will be better equipped to handle the complexities of human language. Integration with other AI technologies, such as voice recognition and image analysis, could further expand its applications and effectiveness.
In conclusion, AI for sentiment prediction is revolutionizing the way we understand and interact with textual data. Its applications span across various industries, providing valuable insights that drive informed decision-making. While challenges remain, ongoing advancements in AI and NLP promise to enhance the accuracy and reliability of sentiment prediction. As we continue to refine these technologies, the potential for AI to transform our understanding of human emotions and language is boundless.